特邀报告
特邀报告1:管晓宏
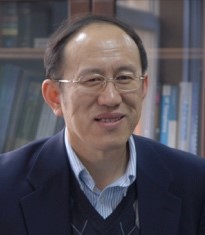
报告讲者:管晓宏
报告时间:2022 年 10 月 29 日 9:10-10:00
报告题目:音乐旋律及其它社会系统中的定量规律 (Quantitative Mechanism in Music Melody and Other Social Systems)
报告摘要:音乐曾经是数学的分支。艺术形象思维启发科学创新,科学技术进步推动艺术的发展。对著名的“李约瑟命题”和“钱学森之问”的探讨,既适用于科学也适用于艺术。艺术与科学的交汇促进理工与艺术教育的共同发展。隐藏在优美旋律中的数学物理规律,与众多自然、工程和社会系统包括语言表达中的定量规律一致,能够定量分析,对艺术创作产生重要影响。报告讨论音乐旋律的三个数学特征,由此建立数学模型,揭示作曲家追求旋律变化的有约束熵最大,从而求解得到音乐旋律变化的幂律。研究结果有助于深度分析音乐艺术特别是作曲理论中的计算智能,探索人工智能辅助作曲的定量化方法。
个人简介:管晓宏,中国科学院院士,IEEE Fellow,分别于1982、1985年获清华大学自动化系学士与硕士学位,1993年获美国康涅狄格大学电气与系统工程系博士学位;1993-1995年任美国PG&E公司高级顾问工程师,1999-2000年任哈佛大学访问科学家,1995年起任西安交通大学教授,1999-2009年任机械制造系统工程国家重点实验室主任,2009-2019年任电子与信息工程学院院长,2019年起任电子与信息学部主任;自2001年先后任清华大学讲席教授组成员、双聘教授,2003-2008年任清华大学自动化系主任;目前兼任中央音乐学院教授和博士生导师。
管晓宏院士主要从事复杂网络化系统的经济性与安全性,电力、能源、制造系统优化,网络信息安全,信息物理融合系统等领域的研究,同时开展作曲理论分析和音乐智能信息处理的研究;曾获2005年、2018年国家自然科学二等奖,2019年何梁何利科技进步奖及多项国际学术奖励。
近年来,管晓宏院士与西安音乐学院艺术学科和交响乐团合作,创办了“艺术与科学的交汇”系列音乐会,采用演奏穿插学术报告形式,以中英文两个版本,先后为清华大学、香港理工大学等10余所境内外高校,全国科技工作者日,省市专场,丝路大学联盟校长和2017 IEEE自动化科学与工程国际年会演出,探讨艺术与科学的关系、音乐中的科学规律,艺术思维对科技创新的启发。管晓宏院士担任音乐会策划和讲解,并与专业交响乐团合作演奏。新近创办的“艺术与科学的交汇”中学版音乐会,从艺术思维与科学思维相互启发的独特维度,促进拔尖创新人才的培养。
特邀报告2:马维英
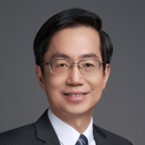
报告讲者:马维英
报告时间:2022 年 10 月 29 日 10:20-11:10
报告题目:Protein as a Foreign Language: Bridging Biomedical Computing and Natural Language Processing
报告摘要:Recently, the rapid development of natural language processing has greatly boosted the progress of other research fields. The impact is especially seen in the field of biological computing, which aims at performing computation on biologically derived molecules, such as DNA and proteins. For instance, the well-known Alpha Fold 2, which employs Transformer-embedded end-to-end learning, has achieved breakthroughs in protein structure prediction. Proteins, sequences of discrete symbols (amino acid), cannot be "read" or "written" by human beings. But each protein describes a unique sequence of "semantics" in the context of life sciences and can be considered as a special kind of language derived from natural evolution. Could such a language difficult for humans to understand be equally inaccessible for machines? In this talk, we will discuss the similarities and differences between human and protein languages, and demonstrate some applications to protein language processing, such as targeted medicine development and antibody neutralization prediction against COVID-19.
个人简介:马维英,清华大学惠妍讲席教授、智能产业研究院首席科学家。他的研究方向包括人工智能的几个核心领域(搜索与推荐、大数据挖掘、机器学习、自然语言理解与生成、计算机视觉)以及人工智能在生命科学、生物制药、基因工程和个体化精准医疗等领域的跨学科研究与应用。他此前曾任字节跳动副总裁兼人工智能实验室主任、前微软亚洲研究院常务副院长。马教授曾在世界级会议和学报上发表过逾 300 篇论文,并拥有160多项技术专利。他是电气电子工程师学会会士(IEEE Fellow),曾任国际信息检索大会(SIGIR 2011)联合主席、国际互联网大会(WWW 2008)的程序委员会联合主席。他于2017年获得吴文俊人工智能科学技术奖二等奖,并曾入选Guide2Research 2018年计算机科学领域TOP100科学家,全球排名86。
特邀报告3:文继荣
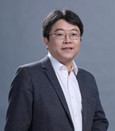
报告讲者:文继荣
报告时间:2022 年 10 月 29 日 11:10-12:00
报告题目:预训练大模型与信息检索
报告摘要:预训练大模型如何与信息检索结合是一个新的开放问题,我将介绍我们在该方向的初步探索,包括通过预训练模型得到语义更为丰富的表示并用于改善检索的各个环节;如何进行专门面向检索任务的预训练;以及以预训练模型为核心的新检索范式。
个人简介:文继荣,教授,现任中国人民大学信息学院院长、高瓴人工智能学院执行院长。长期从事大数据和人工智能领域的研究工作,担任国际会议SIGIR 2020程序委员会主席、国际期刊ACM TOIS和IEEE TKDE编委等。曾任微软亚洲研究院高级研究员和互联网搜索与挖掘组主任。到中国人民大学工作后,积极致力于推动人民大学人工智能和大数据的研究和教学,特别是新技术与相关学科的交叉。2013年入选国家“海外高层次人才计划”特聘专家,2018年入选首批“北京市卓越青年科学家”,2019年担任北京智源人工智能研究院首席科学家。
特邀报告4:杨红霞
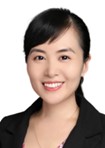
报告讲者:杨红霞
报告时间:2022 年 10 月 30 日 8:30-9:20
报告题目:超大规模多模态预训练模型研发实践和落地应用
报告摘要:近年来,随着预训练技术在深度学习领域的飞速发展,超大规模模型逐渐走进人们的视野,成为人工智能领域的焦点。继OpenAI推出1750亿参数的GPT-3模型之后,我们于自2021年初提出百亿参数的超大规模中文多模态预训练模型M6 (Multi-Modality to Multi-Modality Multitask Mega-transformer),在多项多模态和自然语言下游任务表现出突出的能力。作为业界最大的中文多模态预训练模型M6,我们持续推出多个版本,参数逐步从百亿规模扩展到十万亿规模,在大模型、绿色/低碳AI、AI商业化、服务化等诸多方面取得突破性进展,比如对比相同参数规模1750亿的GPT-3模型,我们只需要其1%的算力,绿色/低碳是大模型普及的必要条件。M6服务内部近50个部门并在阿里云对外200+产品中投入使用,经历过PB级数据的实际检验,同时利用其能力支持多个行业实现创新产品的孵化,如AI服饰设计和数字人。
今年,在探索算力极限的同时,我们也积极展开了针对通用模型这一预训练技术“皇冠”的探索,提出业界首个通用的统一大模型(模态、任务和架构)M6-OFA,极大的降低模型在预训练、适配下游任务、推理过程中的难度,更加便捷的从在线模型构建、在线模型部署、应用发布的全流程预训练服务,能够支持成百上千个应用的开发与部署。同时随着移动芯片计算能力的指数级增长,智能移动设备在内容展示终端这一传统角色之外,逐渐承担起更多任务。如何充分利用好移动算力,我们也探索了一条大模型由云计算走向端计算,端云协同建模M6-Edge。
个人简介:杨红霞,美国杜克大学博士,浙江大学兼职教授,前阿里巴巴达摩院人工智能科学家。主导阿里下一代人工智能突破性技术-认知智能的技术发展与场景应用落地,带领团队研发了AliGraph、M6、洛犀等人工智能开源平台和系统,发表顶级会议、期刊文章近100篇,美国和中国专利20余项。曾获2019年世界人工智能大会最高奖卓越人工智能引领者(Super AI Leader,简称SAIL奖),2020年国家科学技术进步奖二等奖和杭州市领军型创新团队,2021年电子学会科学技术进步奖一等奖,2022年福布斯中国科技女性50。加盟阿里前,曾任IBM全球研发中心Watson研究员, Yahoo!计算广告主管数据科学家。
特邀报告5:Trevor Cohn
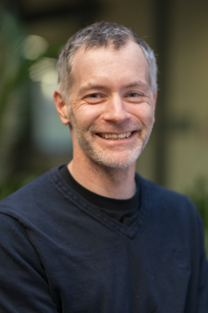
报告讲者:Trevor Cohn
报告时间:2022 年 10 月 30 日 13:30-14:20
报告题目:Is machine translation vulnerable to attack?
报告摘要:Modern advances in natural language processing are based on learning from large text corpora, which are often acquired from myriad online sources such as web news, wikis, blogs and social media. This is especially true for machine translation systems, which require vast collections of parallel bilingual text (sentences and their translations) as well as monolingual texts to produce accurate translation. Accordingly, web scraping is a critical component in leading machine translation systems. This extensive use of online resources, the majority of which are not manually vetted, raises the question of the quality of these resources and the corresponding possibility that systems trained over this data may be compromised by such poor-quality data. More worryingly, the use of untrusted data sources may make trained models vulnerable to specific attacks by a malicious adversary.
In this talk I will report on research on attacks which cause a machine translation model to produce specific incorrect translations given a specific input phrase. These attacks have many worrying potential applications, including phishing, product promotion, spreading misinformation, and defamation. I propose a means of attack based on crafting poisoned parallel or monolingual instances to be incorporated into training resources for a victim system. The attacks exploit known problems with back-translation models, as used in training state of the art neural machine translation systems. Despite the machine translation having many stages to its pipeline, I show that each stage is vulnerable to attack. Overall, the attacks are effective, only requiring a tiny number of poisoned sentences in training to compromise a trained model, and that the attacks are particularly effective against modern large neural architectures for translation, such as the transformer as used in state-of-the-art systems. The last part of the talk will address several defences for translation, and more broadly to related backdoor attacks on text classification models.
个人简介:Dr. Trevor Cohn is a Professor at the University of Melbourne, in the School of Computing and Information Systems. His research focuses on probabilistic and statistical machine learning for natural language processing, with applications ranging from machine translation and multilingual learning to parsing and information extraction. Current projects include measuring and countering demographic biases in text corpora and NLP systems, adversarial attacks on machine translation, and developing corpora and tools for low resource languages. Dr. Cohn has more than 150 research publications, and his research has been recognised by several awards at ACL (2020, 2017) and EMNLP (2016). He served as a local chair for ACL 2018, and as a programme chair for EMNLP in 2020. He received Bachelor degrees in Software Engineering and Commerce, and a PhD degree in Engineering from the University of Melbourne. He was previously based at the University of Sheffield, and before this worked as a Research Fellow at the University of Edinburgh.