第二十届中国计算语言学大会(CCL 2021)特邀报告
特邀报告1:徐宗本
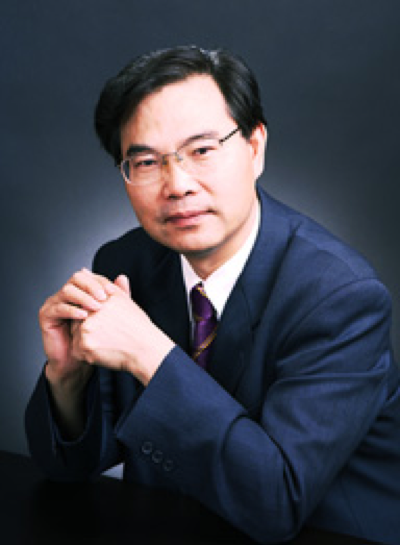
讲者:徐宗本
题目:如何突破机器学习的先验假设?
时间:2021年12月4日09:30-10:30
摘要:机器学习是人工智能的最基础、最核心技术(算法),但机器学习的执行通常都是以一组基本的先验假设为前提的,这些基本假设包括: 假设空间的大容量假设、训练数据的完备性假设、损失度量的数据独立假设、正则项的凭经验设置假设、分析框架的欧氏空间假设等。本报告分析这些假设的作用、局限及其影响,提出突破这些基本假设的可能途径与方法。特别,我们提出突破假设空间大容量假设的模型驱动深度学习方法、突破训练数据完备性假设的课程-自步学习方法、突破损失度量数据独立假设的误差建模原理、突破正则项经验设置假设的隐正则化方法、突破分析框架欧氏空间假设的Banach空间几何方法。每一情况下,我们举例说明新突破带来新价值。所有这些尝试构成机器学习的适配性理论,是当下机器学习研究的一个新方向。
简介:中国科学院院士,数学家、信号与信息处理专家、西安交通大学教授。主要从事智能信息处理、机器学习、数据建模基础理论研究。曾提出稀疏信息处理的L(1/2)正则化理论,为稀疏微波成像提供了重要基础;发现并证明机器学习的“徐-罗奇”定理, 解决了神经网络与模拟演化计算中的一些困难问题,为非欧氏框架下机器学习与非线性分析提供了普遍的数量推演准则; 提出基于视觉认知的数据建模新原理与新方法,形成了聚类分析、判别分析、隐变量分析等系列数据挖掘核心算法, 并广泛应用于科学与工程领域。曾获国家自然科学二等奖、国家科技进步二等奖、陕西省最高科技奖; 国际IAITQM 理查德.普莱斯(Richard Price)数据科学奖; 中国陈嘉庚信息技术科学奖、中国CSIAM苏步青应用数学奖;曾在2010年世界数学家大会上作45分钟特邀报告。
曾任西安交通大学副校长,现任人工智能与数字經济广东省实验室(琶洲实验室)主任、西安数学与数学技术研究院院长、陕西国家应用数学中心主任、大数据算法与分析技术国家工程实验室主任,是国家大数据专家咨询委员会委员、国家新一代人工智能战略咨询委员会委员。
特邀报告2:陶大程
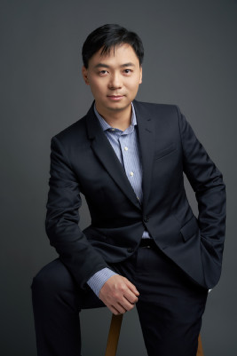
讲者:陶大程
题目:(Re-)building Trust in Deep Learning
时间:2021年12月4日10:30-11:30
摘要:The world is on the eve of the enthusiasm revolution by deep learning sweeping across almost all sectors of our society. Concerns are rising when the deployment has happened in the security critical domains, including autonomous vehicles and medical diagnosis. Fatal disasters on road, infamous privacy breaches, and shocking discrimination scandals undermine public confidence in deep learning applications. In this talk, we will present our perspectives, theory, and practice in (re-)building trust in deep learning.
简介:Dacheng Tao is the President of the JD Explore Academy and a Senior Vice President of JD.com. He is also an advisor of the digital science institute in the University of Sydney. He mainly applies statistics and mathematics to artificial intelligence and data science, and his research is detailed in one monograph and over 200 publications in prestigious journals and proceedings at leading conferences. He received the 2015/2020 Australian Eureka Prize, the 2018 IEEE ICDM Research Contributions Award, and the 2021 IEEE Computer Society McCluskey Technical Achievement Award. He is a fellow of the Australian Academy of Science, AAAS, ACM and IEEE.
特邀报告3:刘嘉
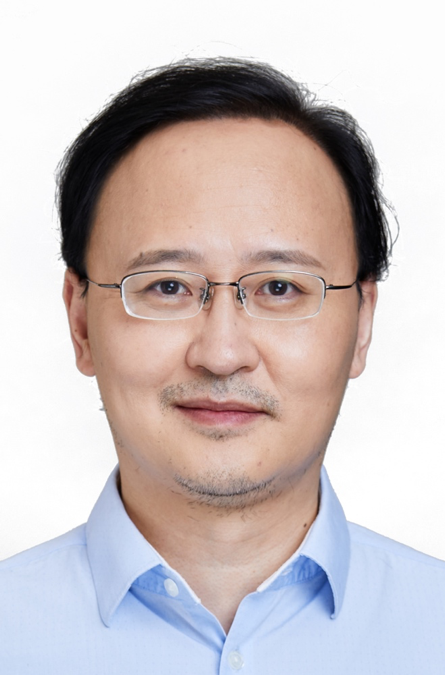
讲者:刘嘉
题目:Principles that govern the functionality of human visual cortex: a perspective from spatial cognition
时间:2021年12月5日09:00-10:00
摘要:Spatial cognition is concerned with the acquisition, organization and utilization of spatial information. It lays the foundation of many other cognitive functions, sets the framework of the mental representation of the world, and is part of core knowledge system on which human intelligence depends. Therefore, the investigation of spatial cognition is critical in revealing the nature of human intelligence. Building upon recent advances in cognitive neuroscience, imaging genetics, developmental psychology and computational modelling, the present study investigates the genetic, neural and behavioral mechanisms of human spatial cognition in particular, and proposes a computational framework on human mental spaces for object recognition and language processing in general that relies on the mechanism of spatial cognition.
简介:刘嘉,清华大学基础科学讲席教授。曾任北师大心理学院院长、心理学部部长,现为清华大学脑与智能实验室首席研究员、北京智源人工智能研究院首席科学家。北京大学心理学系学士、硕士,美国麻省理工学院脑与认知科学系博士。从事心理学/认知神经科学/人工智能的教学、科研和应用工作,是国家杰出青年基金获得者、中科院百人计划入选者、美国富布莱特研究学者、教育部长江学者特聘教授、科技部中青年科技创新领军人才、国家“万人计划”科技创新领军人才、享受政府特殊津贴。先任中国人才学会超常人才专业委员会会长、中国心理学会常务理事、中国高等教育学会常务理事。
特邀报告4:冯胜利
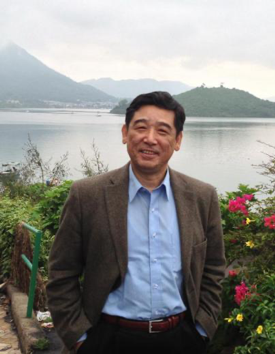
讲者:冯胜利
题目:论语言学理论及其构型条件
时间:2021年12月5日10:30-11:30
摘要:本文从通常情况下对理论的理解和误解入手(如语言=语言学、拿来=理论、技术=理论、说法=理论等等),提出理论构建的学科意义(Yuval Noah Harair 2011),其基本要素包括(但不限于):1、概念(conception)的定义(戴震之“必”、段玉裁之“断无”、以及我们提出的“韵律语法”和“语体语法”等);2、原理(principle)的发掘(如“凡谐声者皆同部”的公理性,声韵相挟必然性);3、溯因(abduction)思维下的领域创设(如相对凸显与心脏跳动→生理节律学[Biometrical phonology]、形式功能对生律与冰锥论→生物物理语体学 [Biophysical Register]等);4、八-tion两段理论构型模式(建筑地基:observation,classification,characterization,generalization;建筑自身:abduction,deduction,prediction,verification)。在此基础之上,文章继而提出理论构型的三个基本条件:(一)理论构建命题为先(命题的句法结构、公理的绝一不二、演绎的族群建立);(二)理论构型必说无(描写说有、理论说无、不说必无难为理论);(三)理论构型必以自家之玉攻它山之石(如四字格、韵律词和韵律句法学的建立)。文章最后指出:中国学术传统自来主张“依自不依他”(章太炎),而当代中国学术则更应承袭乾嘉理必之学,完成从材料归纳到演绎推理的科学转型。
简介:冯胜利,北京语言大学语言科学院教授、博士生导师。美国宾夕法尼亚大学语言学系博士,现任北京语言大学章黄学术理论研究所所长、天津大学语言科学中心首席教授、香港中文大学中国语言及文学系荣誉退休教授。曾任北京语言大学长江学者讲座教授(2005年)、美国堪萨斯大学东亚系副教授,哈佛大学东亚系汉语应用学科教授及中文部主任。其研究兴趣包括乾嘉“理必”与章黄学理研究、训诂学、韵律语法学、语体语法学、汉语历时句法学、汉语韵律文学史。出版学术专著16部(含英文2部,另被译成英文和韩文2部),发表中英文学术论文200余篇。现任《中国语言学报》(JCL,SSCI索引)联席主编和《韵律语法研究》联席主编。
特邀报告5:唐杰
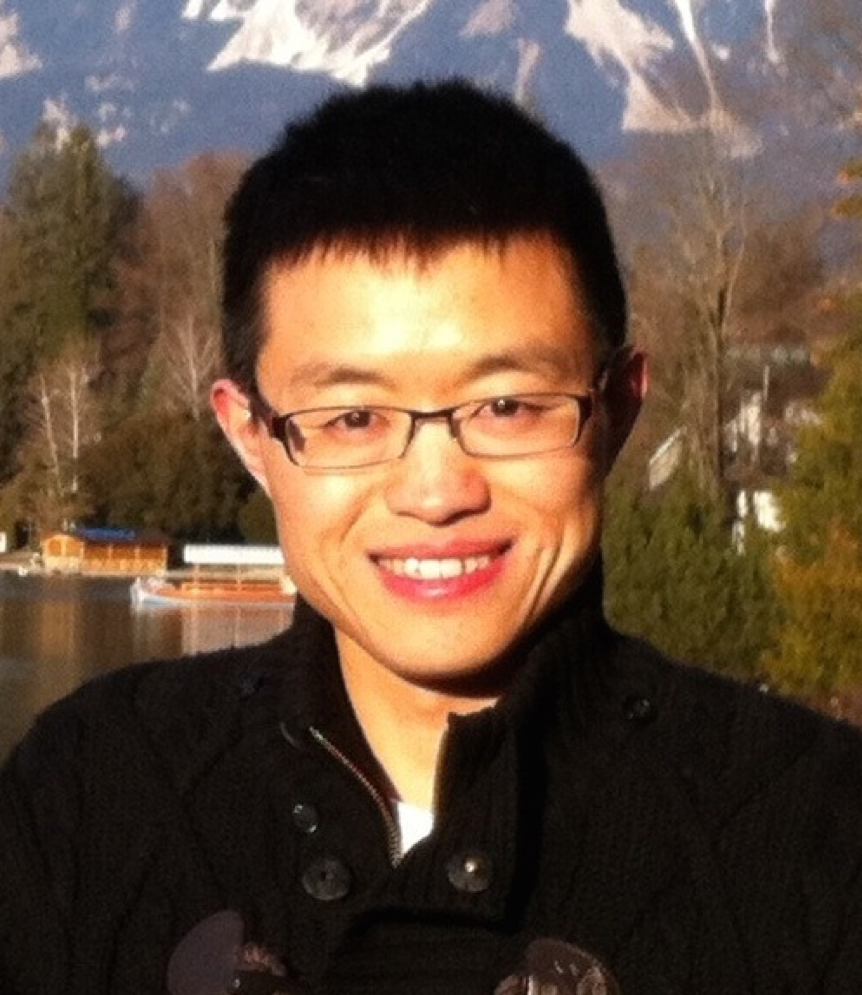
讲者:唐杰
题目:WuDao: Pretrain the World
时间:2021年12月5日13:30-14:30
摘要:Large-scale pretrained model on web texts have substantially advanced the state of the art in various AI tasks, such as natural language understanding and text generation, and image processing, multimodal modeling. The downstream task performances have also constantly increased in the past few years. In this talk, I will first go through three families: augoregressive models (e.g., GPT), autoencoding models (e.g., BERT), and encoder-decoder models. Then, I will introduce China’s first homegrown super-scale intelligent model system, with the goal of building an ultra-large-scale cognitive-oriented pretraining model to focus on essential problems in general artificial intelligence from a cognitive perspective. In particular, as an example, I will elaborate a novel pretraining framework GLM (General Language Model) to address this challenge. GLM has three major benefits: (1) it performs well on classification, unconditional generation, and conditional generation tasks with one single pretrained model; (2) it outperforms BERT-like models on classification due to improved pretrain-finetune consistency; (3) it naturally handles variable-length blank filling which is crucial for many downstream tasks. Empirically, GLM substantially outperforms BERT on the SuperGLUE natural language understanding benchmark with the same amount of pre-training data.
简介:Jie Tang is a Professor and the Associate Chair of the Department of Computer Science at Tsinghua University. He is a Fellow of the IEEE. His interests include artificial intelligence, data mining, social networks, and machine learning. He served as General Co-Chair of WWW’23, and PC Co-Chair of WWW’21, CIKM’16, WSDM’15, and EiC of IEEE T. on Big Data and AI Open J. He leads the project AMiner.org, an AI-enabled research network analysis system, which has attracted more than 20 million users from 220 countries/regions in the world. He was honored with the SIGKDD Test-of-Time Award, the UK Royal Society-Newton Advanced Fellowship Award, NSFC for Distinguished Young Scholar, and KDD’18 Service Award.