Large Model Agents Forum
Host: Chongyang Tao
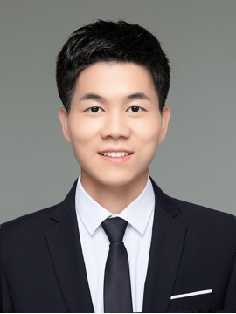
Personal Profile: Chongyang Tao is an Associate Professor at Beihang University. He received his Ph.D. in Science from Peking University in 2020 and later joined Microsoft, where he served as a postdoctoral research scientist and senior research scientist. His research interests include natural language processing and information retrieval, focusing on language models, dialogue systems, efficient knowledge retrieval, among others. He has contributed to the development of Microsoft Xiaobing (Rinna), Bing Chat Assistant, Bing Generation/Search models, and the WizardLM series. He has published over 70 papers in international conferences and journals such as ACL, EMNLP, AAAI, ICLR, SIGIR, and TOIS. He has been awarded the NLPCC Outstanding Paper Award and recognized as an AI 2000 Scholar. He serves as an area chair for conferences like KDD, EMNLP, and CCKS.
Speaker 1: Xu Chen
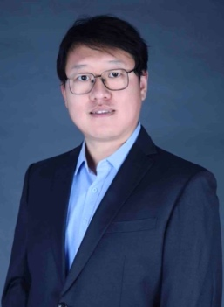
Speaker: Xu Chen
Title: User Behavior Simulation Based on Large Language Model Agents
Abstract: In recent years, Human-centered AI has garnered extensive attention from both academia and industry, with applications such as recommendation systems and social networks greatly facilitating people's lives and productivity. However, a key challenge hindering the development in this field has been acquiring high-quality user behavior data. In this presentation, the speaker will discuss approaches to alleviate this issue from the perspective of LLM-based Agents, and introduce their team's development of RecAgent, an intelligent agent for simulating user behavior based on large language models. This work simulates various behaviors of users in recommendation systems and social networks, where each user acts as an agent capable of engaging in dialogues, posting, searching, self-evolution, etc., within the simulation environment. The speaker will detail the design principles, structural characteristics, usage methods, experimental evaluations of RecAgent, and its potential impacts on the future of Human-centered AI.
Personal Profile: Xu Chen is an Associate Professor at the School of High Ling Artificial Intelligence, Renmin University of China. He received his Ph.D. from Tsinghua University and joined Renmin University of China in 2020. His research focuses on large language models, causal inference, recommendation systems, among others. He has published over 80 papers in top international conferences and journals such as TheWebConf, SIGIR, ICML, NeurIPS, ICLR, AIJ, and KDD, with more than 5800 citations on Google Scholar. He was listed among the top 2% of scientists globally by Stanford University. He co-led the development of the recommendation system toolkit "Bole," co-authored the survey "A Survey on Large Language Model based Autonomous Agents," and constructed the user behavior simulation environment "RecAgent" based on LLM Agents. His research has won the Best Paper Nomination at TheWebConf 2018, the Best Resource Paper Award at CIKM 2022, the Best Paper Nomination at SIGIR-AP 2023, and the Best Paper Award at AIRS 2017. And he has received honors including the CCF Natural Science Second Prize (2nd place), ACM-Beijing Rising Star Award, and CAAI-BDSC Social Computing Young Scholar Rising Star Award. He has led/participated in over ten projects funded by the National Natural Science Foundation of China, the Ministry of Science and Technology, and collaborated with enterprises, with related outcomes implemented in multiple companies. He has been awarded the Huawei "Innovation Pioneer President Award" and the Huawei Excellent School-Enterprise Cooperation Project Award.
Speaker 2: Peng Li
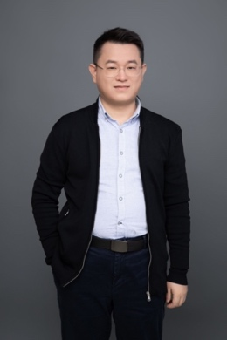
Speaker: Peng Li
Title: Large Model Agents for Open Domain
Abstract: Large models have brought disruptive innovation to the development of artificial intelligence. Effectively utilizing large models to address open-domain issues has become a key topic for the next phase of their development. Recent academic research and industrial practices indicate that intelligent agents based on large models (referred to as large model agents) represent a crucial technological path for extending large models to open domains, with significant research and application prospects. This presentation will share and discuss the main challenges, innovative approaches, and future development directions for large model agents in open domains.
Personal Profile: Peng Li is an Associate Researcher/Associate Professor at the Institute for AI Industry Research, Tsinghua University. His main research interests include natural language processing, pre-trained language models, cross-modal information processing, and large model agents. He has published over 90 papers at major international AI conferences and journals, received the ACL 2023 Outstanding Paper Award, and topped several internationally influential leaderboards, surpassing teams from Google Research, OpenAI, and others. He has led projects such as the key topic under the Science and Technology Innovation 2030 Major Project and the General Program of the National Natural Science Foundation of China. He has served as area chair for important international conferences such as NAACL, COLING, EACL, and AACL. His research outcomes have been applied to high-daily-active-user products from Baidu and Tencent WeChat, achieving significant impact and earning the First Prize of the Qian Weichang Chinese Information Processing Science and Technology Award from the Chinese Information Processing Society of China.
Speaker 3: Xin Gao
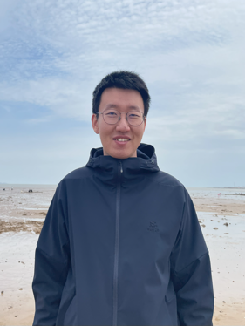
Speaker: Xin Gao
Title: Tool Learning for Large Model-based Agents
Abstract: Research on agents based on large-scale language models is an emerging direction in the field of natural language processing, further advancing the development of general artificial intelligence. This presentation will focus on building the tool invocation capabilities of language model agents, exploring methods for developing foundational tool-use skills in language model agents, and how these can be applied to more downstream tasks.
Personal Profile: Xin Gao is a Distinguished Researcher and Ph.D. Supervisor at the School of Computer Science, University of Electronic Science and Technology of China. His main research areas are pre-trained language models, large model agents, and tool learning. He has published over 40 papers in top international conferences and journals. He currently serves as a member of the Youth Working Committee of the Chinese Information Processing Society of China and a communications member of the Information Retrieval Committee. He is also an area chair and senior program committee member for several top conferences.
Speaker 4: Chen Qian
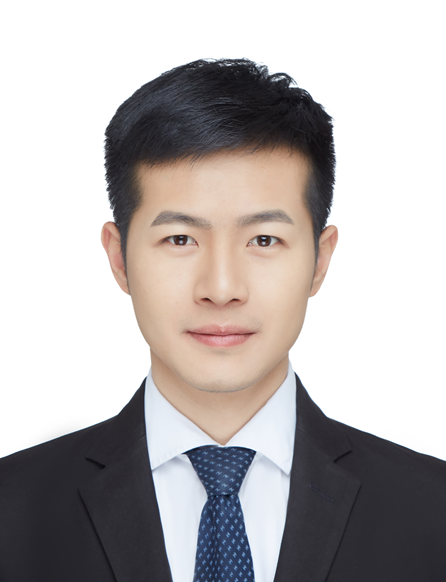
Speaker: Chen Qian
Title: Preliminary Exploration of Scaling Laws for Collaborative Large Model Agents
Abstract: Contemporary large model-driven group collaboration aims to create a virtual team of multiple collaborative agents, autonomously generating complete solutions based on specific task requirements posed by human users through interactive collaboration. This approach achieves efficient and economical reasoning processes, providing new possibilities for automating complex problem-solving. The relevant technology is expected to effectively free humans from traditional labor, realizing the vision of "agents assisting human work." This presentation will cover the key technologies of collaborative multi-agent systems based on large models, including advancements in interaction, collaboration, and evolution, and will preliminarily explore scaling laws for collaboration to guide the construction of efficient multi-agent systems.
Personal Profile: Chen Qian holds a Ph.D. from the School of Software, Tsinghua University, and is currently a postdoctoral researcher at the Tsinghua University Natural Language Processing Lab (THUNLP) and a Shuimu Scholar at Tsinghua University. His main research areas are pre-trained models, autonomous agents, and swarm intelligence. His co-advisors are Professors Maosong Sun and Zhiyuan Liu. He has published several papers as the first author in international academic conferences and journals related to artificial intelligence, information management, and software engineering, such as ACL, SIGIR, ICLR, AAAI, and CIKM. In the field of swarm intelligence, he has led the release of the large language model-driven group collaboration framework ChatDev, the group co-learning paradigm Co-Learning, and the group collaboration network MacNet. He also contributed to the development of the multi-agent platform AgentVerse for task completion and social simulation. ChatDev has gained over 20,000 stars on GitHub, receiving high praise from renowned scholars and enterprises worldwide. Andrew Ng, one of the most authoritative scholars in AI and machine learning, highlighted ChatDev as a representative case in his latest trends and insights on agents published in March 2024.
Speaker 5: Haifeng Zhang
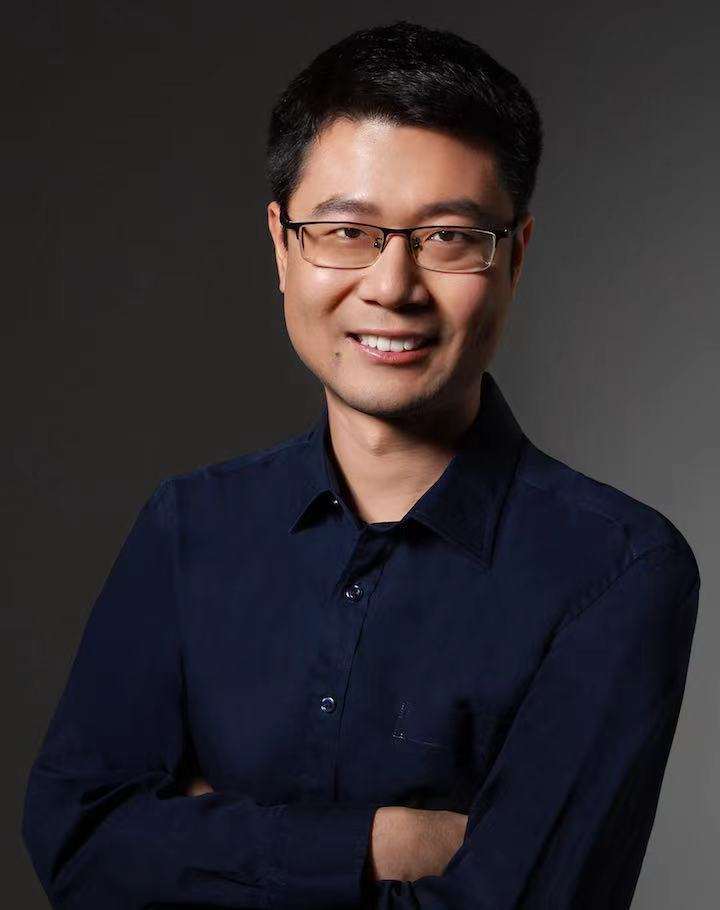
Speaker: Haifeng Zhang
Title: Game Agents Driven by Large Language Models
Abstract: Game agents are a significant thread in the development of artificial intelligence. The advent of large language models provides a new approach to constructing game agents. By using large language models as a foundation and integrating specialized game strategies, it is possible to build game agents with certain general capabilities at a relatively low cost. This presentation will explore the application of this method in various virtual and real-world game scenarios, including StarCraft, soccer games, and socio-economic environments.
Personal Profile: Haifeng Zhang is an Associate Researcher at the Institute of Automation, Chinese Academy of Sciences, and the leader of the Group Decision Intelligence team. He received his bachelor's and doctoral degrees from the Department of Computer Science at Peking University and has conducted postdoctoral research at University College London (UCL). His work focuses on academic research and platform development for multi-agent systems and reinforcement learning. His papers have been published in renowned academic conferences and journals such as ICML, IJCAI, AAAI, AAMAS, and Journal of Software. He leads the development of the "Jidi" intelligent game platform (www.jidiai.cn) at the Institute of Automation, Chinese Academy of Sciences, and undertakes several projects including the National Natural Science Foundation, the Ministry of Science and Technology's "New Generation Artificial Intelligence" major project, and the Chinese Academy of Sciences Pioneer A project. His research has been applied in various fields, including game agents, oil and gas industry chain scheduling, and railway timetable adjustments.
Speaker 6: Ningyu Zhang
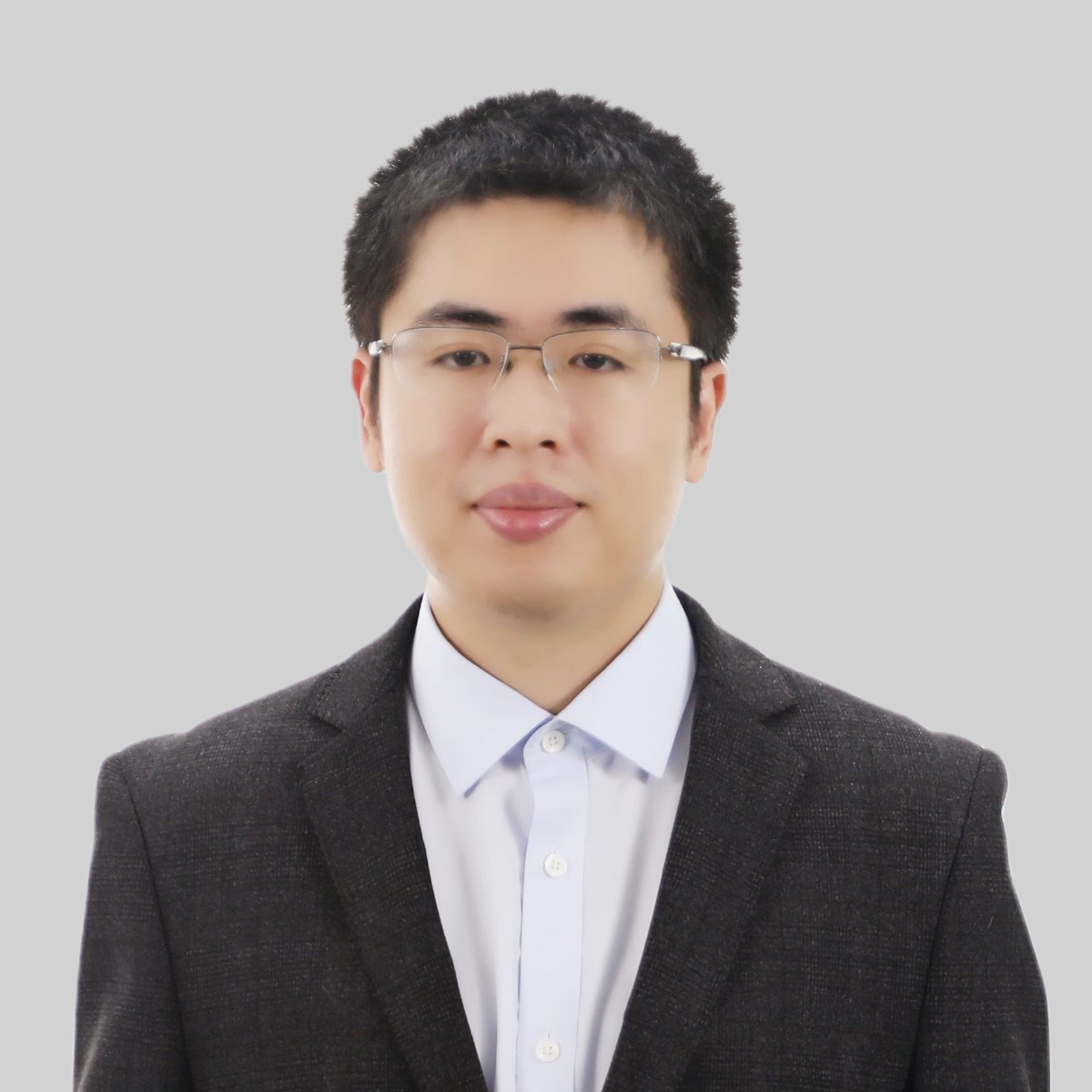
Speaker: Ningyu Zhang
Title: Intelligent Agent Evolution from the Perspective of Knowledge Editing
Abstract: The evolution of large model intelligent agents is a process of enhancing their capabilities through continuous accumulation and optimization of knowledge. In this process, agents improve their knowledge base and decision-making abilities through interaction, learning, and self-improvement. This presentation will explain the process of memory updating and capability evolution in intelligent agents from the perspective of knowledge editing. It will also introduce work related to symbolic and parametric knowledge enhancement for agents. Finally, it will discuss the potential of continuously correcting and expanding the knowledge structure of agents through knowledge editing operations, enabling them to maintain adaptability and flexibility in dynamic environments and better understand complex tasks to improve problem-solving abilities.
Personal Profile: Ningyu Zhang is an Associate Professor at Zhejiang University and a Qi Zhen Excellent Young Scholar at Zhejiang University. He has published numerous papers in high-level international academic journals and conferences, with six papers selected as high-impact papers by Paper Digest and one featured as a Featured Article in a Nature sub-journal. He has led multiple projects funded by the National Natural Science Foundation, the China Computer Federation, and the Chinese Association for Artificial Intelligence. He has received the Second Prize for Scientific and Technological Progress in Zhejiang Province, the IJCKG Best Paper Award/Nomination twice, and the CCKS Best Paper Award once. He has served as Area Chair for ACL and EMNLP, an Action Editor for ARR, and a Senior Program Committee Member for IJCAI. He has led the development of the large language model knowledge editing tool EasyEdit (1.6k).