Invited Talk
Invited Talk 1: AI Approaches Driven by the Dual Engines of Large Models and Large Databases(Academician Weinan E, Peking University)
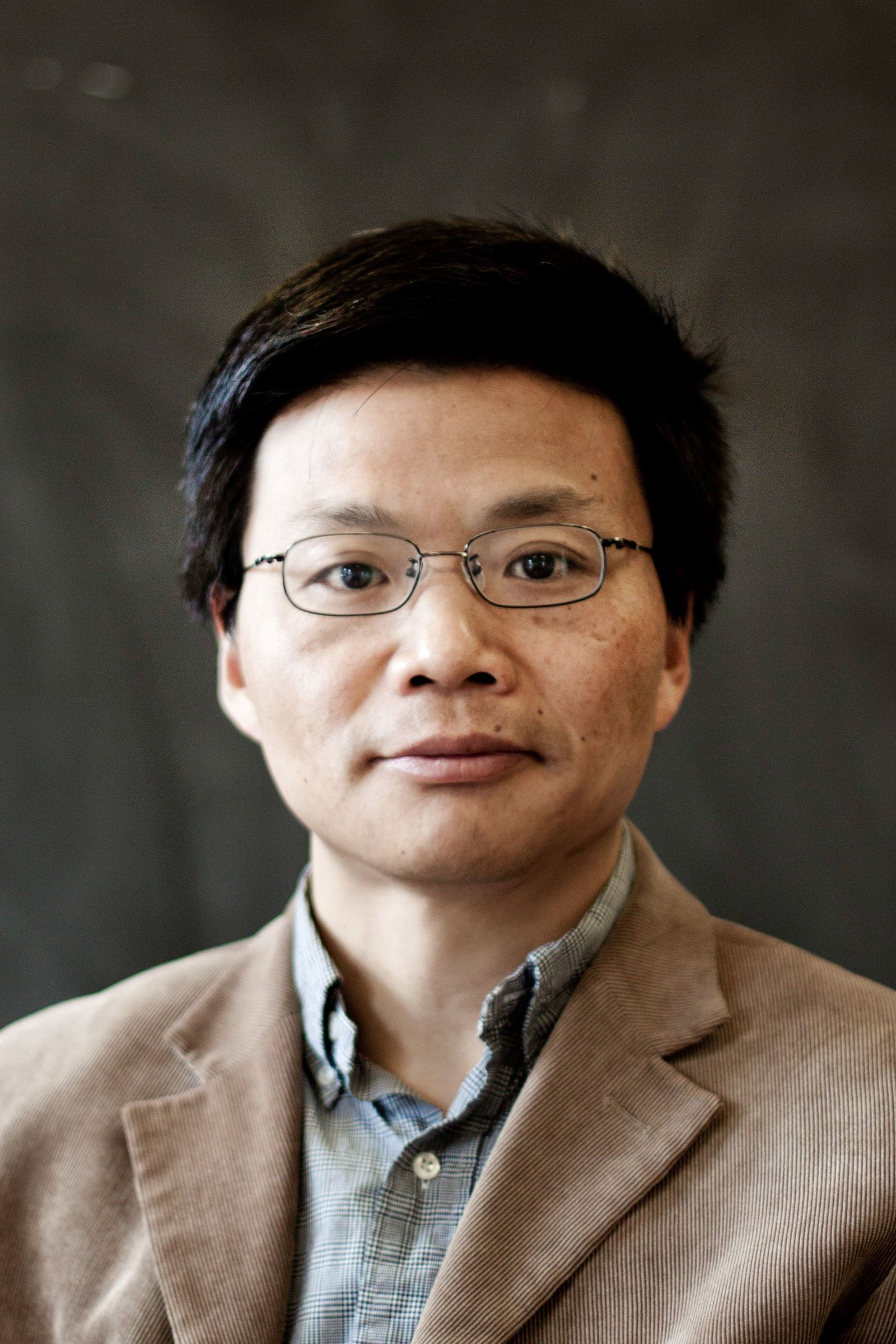
Speaker: Academician Weinan E (Peking University)
Title: AI Approaches Driven by the Dual Engines of Large Models and Large Databases
Abstract: In this presentation, we will focus on how to combine the methods of large models with high-performance general AI database methods to establish efficient, accurate, low-threshold, and cost-effective artificial intelligence systems.
Personal Profile: Weinan E is a professor in the Center for Machine Learning Research (CMLR) and the School of Mathematical Sciences at Peking University. He is also the inaugural director of the AI for Science Institute in Beijing, as well as the director of the Beijing Institute for Big Data Research. He is a member of the Chinese Academy of Sciences; a fellow of SIAM, AMS, IOP, CSIAM,ORSC and CCF.
His main research interest is numerical algorithms, machine learning and multi-scale modeling, with applications to chemistry, material sciences and fluid mechanics. He was a plenary speaker at the 2022 International Congress of Mathematicians (ICM), a keynote speaker at the 2022 International Conference on Machine Learning (ICML) and an invited speaker at ICM2002 and ICIAM (International Congress of Industrial and Applied Mathematics) 2007. He has also been invited speaker at leading conferences in many other scientific disciplines, including the APS, ACS, AIChe annual meetings, the American Conference of Theoretical Chemistry and the World Congress of Computational Mechanics.
He was awarded the ICIAM Collatz Prize in 2003, the SIAM Kleinman Prize in 2009, the SIAM von Karman Prize in 2014, the SIAM-ETH Peter Henrici Prize in 2019, the ACM Gordon-Bell Prize in 2020, and the ICIAM Maxwell Prize in 2023.
Invited Talk 2: Research Progress and Prospects in Representation Learning(Professor Jiye Liang, Shanxi University)
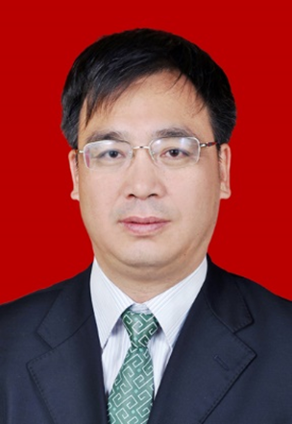
Speaker: Professor Jiye Liang (Shanxi University)
Title: Research Progress and Prospects in Representation Learning
Abstract:
The performance of machine learning methods heavily relies on data representation. In the era of deep learning, data representation is integrated into the learning process, making the acquisition of good data representation a focal point of learning. Currently, representation learning has become a significant research direction in the fields of machine learning and artificial intelligence. This presentation will first introduce the relevant background, main methods, and key issues of representation learning. Next, it will elaborate on our latest research progress in representation learning from the perspectives of concept cognition, generalization error, and Bayesian error rate. Finally, it will share some thoughts on representation learning to inspire future research.
Personal Profile:
Jiye Liang is a professor and doctoral supervisor at Shanxi University. He is a Fellow of the China Computer Federation (CCF) and the Chinese Association for Artificial Intelligence (CAAI). Dr. Liang serves as the Director of the Academic Committee of Shanxi University and the Director of the Key Laboratory of Computational Intelligence and Chinese Information Processing of the Ministry of Education. He has previously served as Vice President (at the level of university president) of Shanxi University and as Dean of Taiyuan Normal University.
Dr. Liang is currently a member of the Artificial Intelligence and Blockchain Special Committee of the Ministry of Education's Science and Technology Committee and a member of the Education Steering Committee for Computer Science Majors. He is also the Director of the Artificial Intelligence and Pattern Recognition Special Committee of the China Computer Federation and the Chairman of the Shanxi Computer Society. Dr. Liang is recognized as a Expert enjoying a special allowance from the State Council of China.
He has led more than 10 projects including the Science and Technology Innovation "2030—New Generation Artificial Intelligence" Major Project, the National Natural Science Foundation Key Project, and the National 863 Program Project. Dr. Liang has published over 300 papers in international and domestic prestigious academic journals and conferences such as AI, JMLR, IEEE TPAMI, IEEE TKDE, NeurIPS, and ICML. Under his guidance, four doctoral students have respectively won the National Excellent Doctoral Dissertation Nomination Award, the CCF Excellent Doctoral Dissertation Award, the CAAI Excellent Doctoral Dissertation Award, and the Excellent Doctoral Dissertation Award of the Chinese Society of Chinese Information Processing.
Invited Talk 3: Some Reflections on Cognition-Inspired General Artificial Intelligence (Sen Song, Researcher at Tsinghua University)
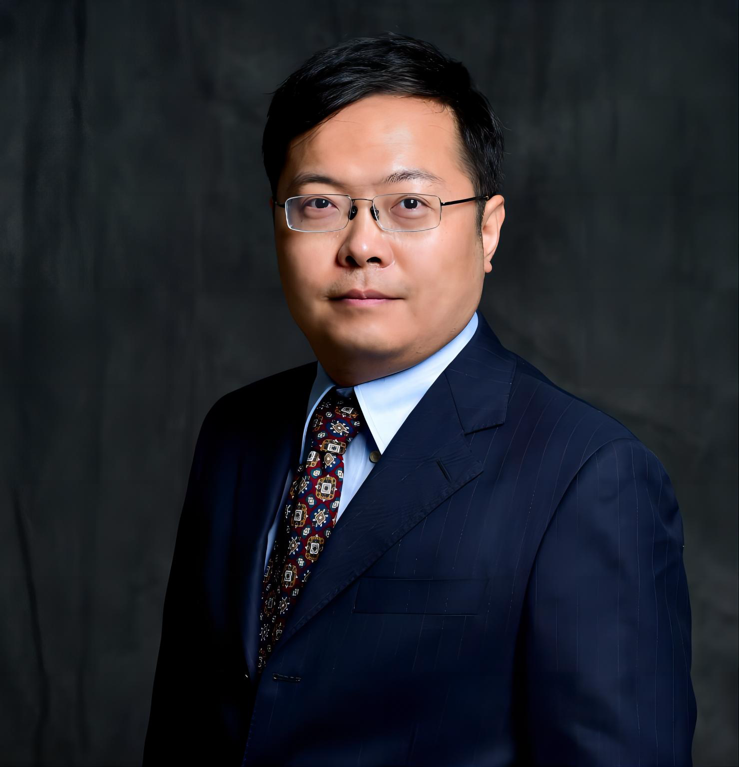
Speaker: Researcher Sen Song (Tsinghua University)
Title: Some Reflections on Cognition-Inspired General Artificial Intelligence
Abstract: Recently, AI driven by large models has made significant advances, but its shortcomings have become increasingly apparent. I will attempt to propose some characteristics that general artificial intelligence may need from the perspectives of cognitive science and neuroscience. Drawing from my research, I will present several cases of cognition-inspired AI, focusing on possible interactions between System 1 and System 2, as well as areas such as rule learning.
Personal Profile:
Sen Song, Tenured Associate Professor at Tsinghua University, Assistant Director of the Brain and Intelligence Laboratory, and Researcher at the School of Biomedical Engineering. He received his Ph.D. in Computational Neuroscience from Brandeis University in 2002 and completed postdoctoral research at Cold Spring Harbor Laboratory and the Massachusetts Institute of Technology. Since joining Tsinghua University in 2010, he has focused on computational neuroscience and brain-inspired intelligence research. For over 20 years, he has been dedicated to the intersection of neuroscience and artificial intelligence, aiming to elucidate the principles of intelligence and apply them to major issues in biology and medicine. He has achieved a series of internationally leading results in brain-inspired computation and intelligence, key theoretical principles, model algorithms, and architectural applications. His research findings have been published in top international conferences and journals such as Nature, NeurIPS, ICML, and ACL, with over 60 papers. One of his papers on spike-timing-dependent plasticity has been cited 3130 times. His Tianjichip paper was published in Nature and was recognized as one of China's Top Ten Scientific Advances of the Year.
Invited Talk 4: Artificial Intelligence: Navigating Between Neural and Symbolic (Qun Liu, Chief Scientist of Speech and Semantics at Huawei)
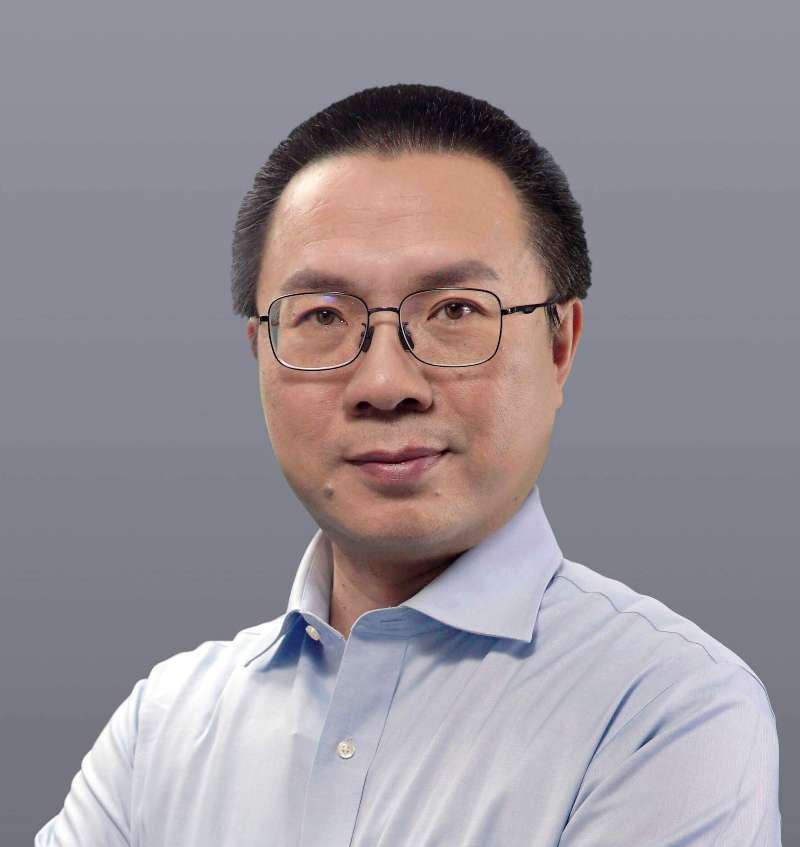
Speaker: Qun Liu, Chief Scientist of Speech and Semantics (Huawei)
Title: Artificial Intelligence: Navigating Between Neural and Symbolic
Abstract: The debate between neural and symbolic approaches in artificial intelligence has a long history. Large language models have pushed the capabilities of neural methods to their limits. Will neural network methods, with large language models at their core, ultimately be able to perfectly simulate human intelligence? Is there still a necessity for symbolic computation in artificial intelligence? This talk aims to systematically organize and discuss this issue. It will first outline the relevant concepts related to the neural-symbolic debate, then discuss the necessity, advantages, and disadvantages of combining neural and symbolic methods. From the perspective of knowledge representation, it will categorize and summarize various methods of integrating neural and symbolic approaches. Finally, it will look ahead to the future development directions in this field.
Personal Profile:
Qun Liu, Professor and Chief Scientist of Speech and Semantics at Huawei, ACL Fellow. Since 2018, he has led the Speech and Semantics team at Huawei Noah's Ark Lab, developing technologies including machine translation, dialogue systems, speech recognition and synthesis, and pre-trained large language models, providing strong support for Huawei's products and services. Prior to joining Huawei, he was a Professor at Dublin City University in Ireland and the leader of the Natural Language Processing theme at the ADAPT Centre starting in 2012. Before that, he worked at the Institute of Computing Technology, Chinese Academy of Sciences (CAS), for 20 years as a Researcher, where he founded and led the Natural Language Processing research group. He received his Bachelor's, Master's, and Ph.D. degrees in Computer Science from the University of Science and Technology of China, the Institute of Computing Technology of CAS, and Peking University, respectively. His main research interests are in natural language processing, with research outcomes including Chinese word segmentation and part-of-speech tagging systems, statistical and neural machine translation, pre-trained language models, question answering, and dialogue systems. He has published over 300 papers in professional conferences and journals, which have been cited more than 19,000 times, and has supervised over 50 Ph.D. and Master's graduates both domestically and internationally. He has received numerous awards, including the Google Research Award, ACL Best Long Paper, Qian Weichang Chinese Information Processing Science and Technology First Prize, National Science and Technology Progress Second Prize, and IAMT Honor Award.
Invited Talk 5: Preliminary Exploration of Large Model Alignment Technology (Xuanjing Huang, Professor at Fudan University)
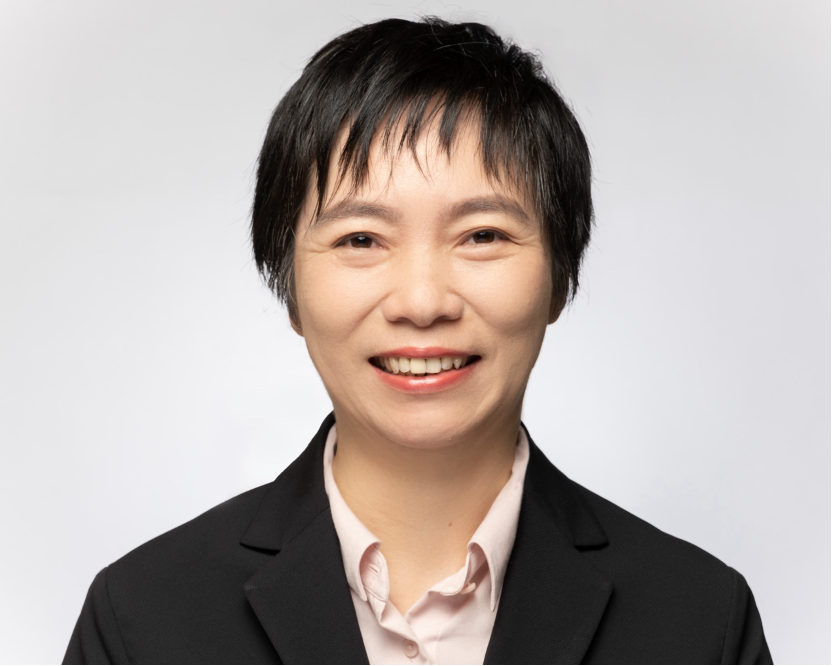
Speaker: Xuanjing Huang, Professor (Fudan University)
Title: Preliminary Exploration of Large Model Alignment Technology
Abstract: Large model alignment refers to the process of optimizing the behavior and output of large models to align with human intentions and ethical values, which is crucial for ensuring the safety and reliability of generative artificial intelligence. This report focuses on the capability and value alignment of large models. It first explores how to use reinforcement learning algorithms based on human feedback, utilizing human preference data to train reward models, and then applying algorithms such as Proximal Policy Optimization (PPO) to embed complex human values and ethical principles into large models to achieve value alignment. Next, it discusses how to enhance large model capabilities from multiple perspectives through human preference learning, ensuring the safety, fairness, and transparency of models when handling complex tasks. Following this, the report introduces dialogue-based and multimodal large models developed by the Fudan University team, sharing insights on effectively applying large models to various real-world scenarios, such as intelligent assistants and multimodal interactions.
Personal Profile:
Xuanjing Huang is a professor at Fudan University and a leading talent in technological innovation under the national "Ten Thousand Talents Plan." Her primary research interests are artificial intelligence, natural language processing, and information retrieval. She serves as a director of the Chinese Information Processing Society of China (CIPSC), chair of the Natural Language Processing Committee of the China Computer Federation (CCF), and vice president of the Asia-Pacific Chapter of the Association for Computational Linguistics (AACL). In recent years, she has led multiple national and provincial-level research projects and published over 200 papers in major international academic journals and conferences, with more than 20,000 citations and eight best paper awards. She has received numerous accolades, including the Qian Weichang Chinese Information Processing Science and Technology Award, Shanghai Outstanding Academic Leader, Shanghai Education Talent Award, Global Women in AI Scholar, and Forbes China Women in Technology.
Invited Talk 6: Understanding and Navigating Human Control and Transparency in Language Models (Ivan Titov, Professor at the University of Edinburgh & University of Amsterdam)
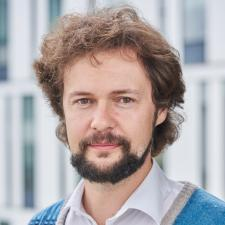
Speaker: Professor Ivan Titov (University of Edinburgh & University of Amsterda)
Title: Understanding and Navigating Human Control and Transparency in Language Models
Abstract: Language models represent an exciting technology that has transformed our field and are now used by millions of people daily. However, both users and researchers often find themselves puzzled by their responses and struggle to understand the underlying decision processes or attribute their responses to specific data sources. Our group's work tries to enhance the transparency of these models for human users, ensure their behavior is systematic, and uncover the sources of their decisions. This transparency should enable finer control of these models, including model editing or the unlearning of undesirable behaviors or data sources.
In this talk, I will discuss the approaches my group and other colleagues have been developing, highlighting not only methods but also some cautious lessons learned along the way. This includes pitfalls in data attribution and the challenges of guiding model responses with human rationale. Although progress in these areas may seem slow and sometimes illusory, it is a crucial direction, given the growing reliance on collaboration between humans and large language models. I also hope to convince you that this area holds a diverse range of intriguing open problems for researchers to explore.
Personal Profile:
Ivan Titov is a Professor at the University of Edinburgh, UK, and a co-opted faculty member at the University of Amsterdam, Netherlands. Ivan's current interests lie in making deep learning models interpretable, robust, and controllable, or more generally in machine learning for NLP. He has received awards at leading NLP conferences. Ivan has been a program co-chair of ICLR 2021 and CoNLL 2018, and has served on the editorial boards of the Transactions of the ACL, Journal of Artificial Intelligence Research, and Journal of Machine Learning Research, and on the advisory board of the European chapter of ACL. Ivan is an ELLIS fellow and co-directs the ELLIS NLP program and Edinburgh ELLIS unit. Ivan's research group has been supported by personal fellowships (e.g., ERC, Dutch Vici, and Vidi grants) as well as industrial funding (e.g., Google, SAP, Booking.com and Amazon).