Student Workshop
Speaker 1: Zhuosheng Zhang
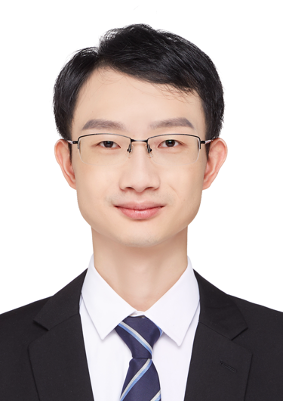
Speaker: Zhuosheng Zhang
Title: How to Sustain Research Motivation in the Era of Large Models?
Abstract: The research paradigm in the era of large models is undergoing profound changes. The roles of industry and academia are continuously evolving, and the standards for evaluating research work have become more diversified. This report will draw on the speaker's research experiences in both corporate and academic settings to explore how to choose appropriate research directions with limited resources in universities and dynamically adjust and upgrade them at different stages of growth. Additionally, the report will share insights on collaborating effectively with mentors and finding unique research paths. It is hoped that students will continuously discover interesting and meaningful research questions, leading to impactful work.
Personal Profile: Zhuosheng Zhang is a tenure-track assistant professor at Shanghai Jiao Tong University. His primary research interests include natural language processing, large models, and security. His notable works include Automatic Chain of Thought Reasoning (Auto-CoT), Multimodal Chain of Thought Reasoning (MM-CoT), Multimodal GUI Agent (Auto-GUI), and Safety Evaluation of Large Model Agents (R-Judge). He has published over 60 papers in top-tier journals and conferences such as TPAMI, ICLR, ICML, ACL, and AAAI, with more than 30 papers as the first or corresponding author. His Google Scholar citations exceed 4,200, and his open-source contributions have garnered over 10,000 stars on GitHub. Zhuosheng Zhang was selected for the 2023 Doctoral Dissertation Award Program by the Chinese Information Processing Society, the 2023 WAIC Yunfan Award Rising Star, and the 2021 Global AI Chinese New Star 100. He has interned or visited institutions including Amazon Web Services, Microsoft Research Redmond, Lanzhou Technology, and the National Institute of Information and Communications Technology (NICT) in Japan.
Speaker 2: Bingning Wang
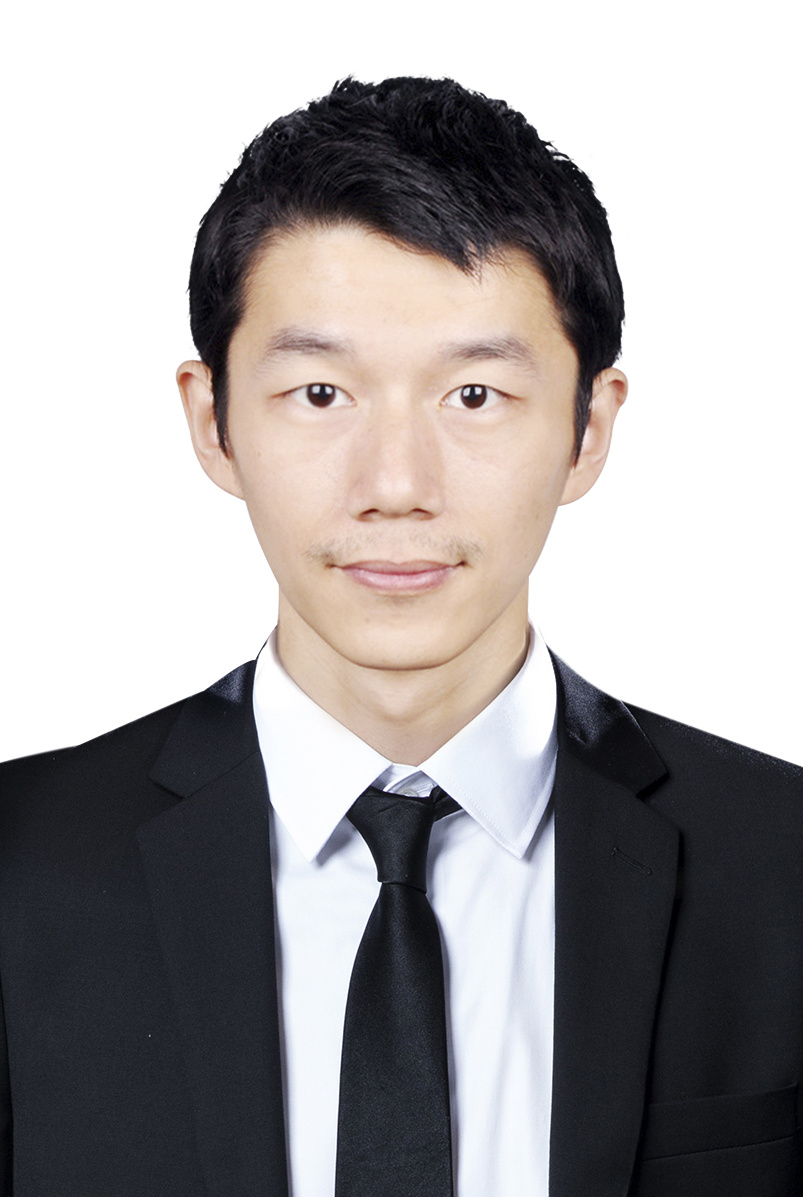
Speaker: Bingning Wang
Title: Enhancing Students' Application Abilities in the Era of Large Models
Abstract: With the advent of the era of large models, the research topics and methods in academia and industry are becoming increasingly similar. This convergence presents significant challenges for young researchers and students who have little experience with practical large model projects. The gap between academic research and industrial research seems to be widening, particularly due to disparities in computing power. This report will explore how students can adapt to these changes in the current era of large models and focus their research on meaningful projects.
Personal Profile: Bingning Wang is the head of pre-training at Baichuan Intelligent. He holds a Ph.D. from the Institute of Automation, Chinese Academy of Sciences, and his primary research areas are question-answering systems and large language models. Bingning has held senior researcher positions at Sogou and Tencent, and has extensive experience with large-scale generative models. He has led and released large-scale Chinese QA datasets such as ReCO, ComQA, ChiQA, and T2Ranking, as well as the Baichuan series of pre-trained models. Bingning has published 11 papers as the first author at top international AI and NLP conferences such as ACL, SIGIR, and AAAI, and received the 2021 CIKM Best Paper Runner-Up Award. His doctoral dissertation, "Key Technologies for Machine Reading Comprehension," won the Outstanding Doctoral Dissertation Award from the Chinese Information Processing Society in 2019. He is also a member of the Youth Working Committee of the Chinese Information Processing Society.
Speaker 3: Pengfei Cao
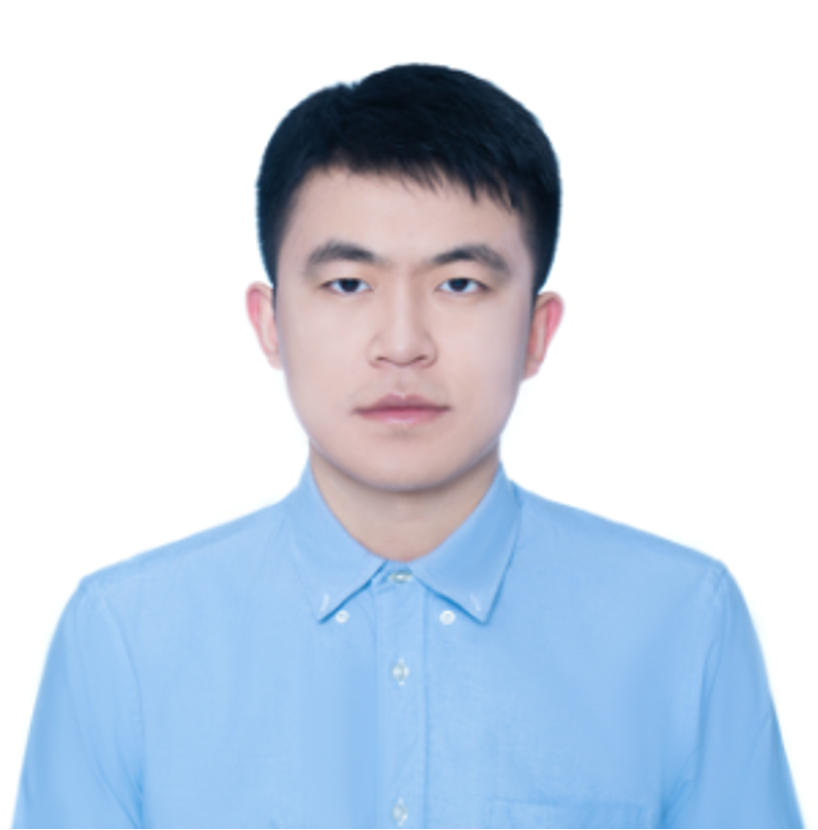
Speaker: Pengfei Cao
Title: The Transition from Student to Young Faculty in Research
Abstract: With the rapid development of large model technology, natural language processing has entered a new historical phase, bringing new opportunities and challenges for researchers. This report will draw on the speaker's experience transitioning from a student to a young faculty member to explore how to adapt to the rapidly changing research environment, adjust research directions, and shift research mindsets to seize emerging research opportunities. The goal of this report is to provide students with insights on how to navigate their research paths and continue pursuing academic research.
Personal Profile: Pengfei Cao is an assistant researcher at the Institute of Automation, Chinese Academy of Sciences. He received his Ph.D. from the Institute of Automation, Chinese Academy of Sciences in 2023. His primary research interests are natural language processing, large language models, and information extraction. In recent years, he has published over 30 papers at major international conferences in AI and NLP, such as AAAI, ACL, EMNLP, and CIKM. He has served as an area chair for ACL and NAACL, and as a reviewer for major journals and conferences including TKDE, AAAI, ACL, and EMNLP. He has received the Special Research Assistant Grant from the Chinese Academy of Sciences, the President's Award of the Chinese Academy of Sciences, and the Outstanding Doctoral Dissertation Award from the Chinese Information Processing Society.
Speaker 4: Kun Zhou
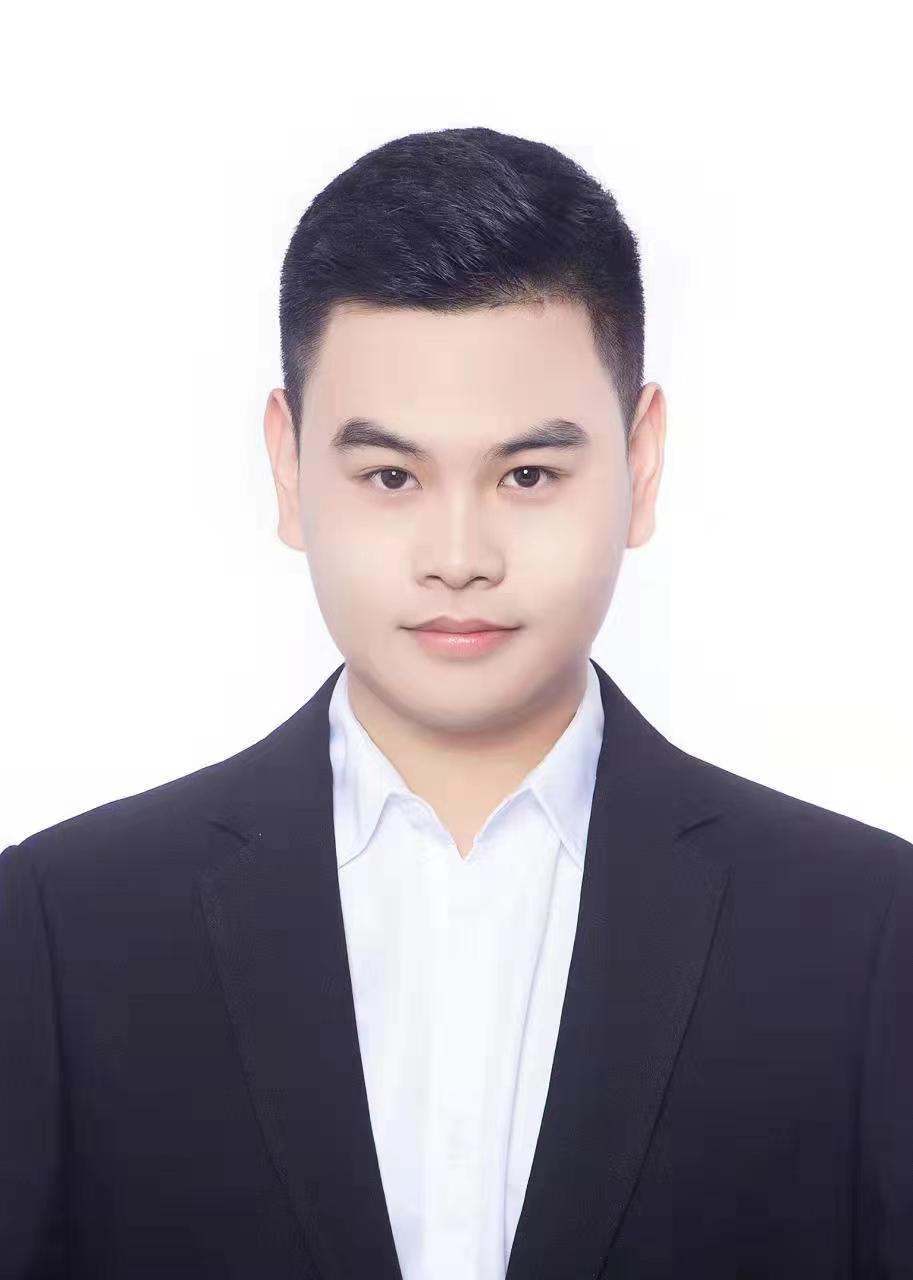
Speaker: Kun Zhou
Title: Experiences of a PhD Student Entering a New Research Field
Abstract: In recent years, the AI community has seen the emergence of many new technologies, which, while revolutionizing the field, also bring immense pressure on researchers to learn new technologies rapidly. This report will present the speaker's personal perspective, using the example of large language models, to illustrate how an ordinary PhD student can quickly enter a new research field. It aims to provide a reference for students experiencing similar anxieties.
Personal Profile: Kun Zhou is a PhD student (class of 2020) at the School of Information, Renmin University of China, under the supervision of Professors Jirong Wen and Xin Zhao. His research focuses on large language models, natural language processing, and information retrieval. To date, he has published over ten papers as the first author at top conferences in the field, with over 4,000 citations. He has received several honors including the EACL 2024 Evaluation and Model Insight Award, the 2022 National Scholarship, the 2022 Baidu Scholarship, the 2022 ByteDance Scholarship, and the 2022 Microsoft Scholar Award.
Speaker 5: Fanghua Ye
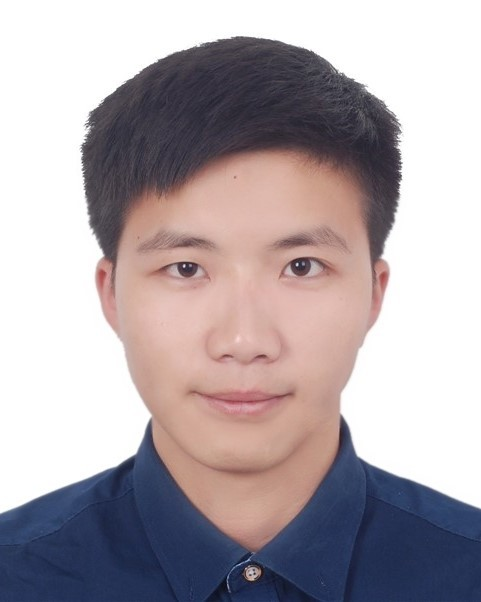
Speaker: Fanghua Ye
Title: Insights from Pursuing a PhD in the UK
Abstract: The UK, as a longstanding educational powerhouse, attracts numerous international students every year. In this talk, I will share my experiences and insights from pursuing a PhD in the UK, covering various aspects such as the application process, the UK education system, academic environment, student-supervisor relationships, cultural differences, social life, and job preparation. I hope my sharing will provide useful references for students interested in studying in the UK.
Personal Profile: Fanghua Ye is a PhD student at University College London, supervised by Professors Emine Yilmaz and Jun Wang. His main research interests are dialogue systems, large language models, and information retrieval. In recent years, he has published several papers at international conferences such as ACL, EMNLP, WWW, NeurIPS, ICDM, and SIGMOD, and served as an area chair for EMNLP 2023.
Speaker 6: Shikun Feng
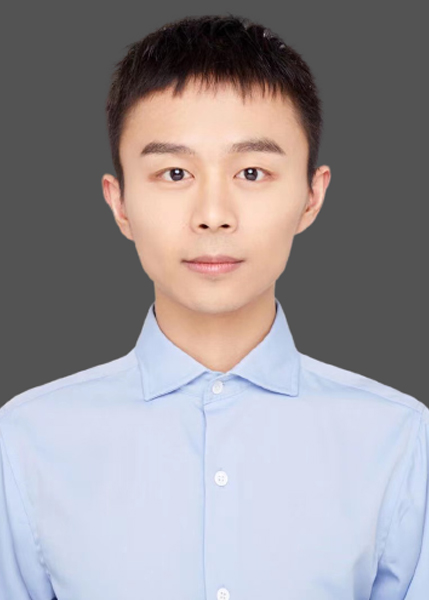
Speaker: Shikun Feng
Title: Physical informed molecule Pre-training
Abstract: Molecule pre-training is a crucial technology in drug discovery, as the learned molecular representations can be applied to various downstream tasks related to molecular property prediction. Most existing molecule pre-training methods are directly adapted from the NLP and CV fields, lacking explanations for the chemical and physical properties of the molecules themselves. To address this, we propose a series of pre-training methods inspired by molecular physical properties, Frad and SliDe, which learn and characterize the force fields of molecules during pre-training. Experiments demonstrate that our methods achieve state-of-the-art performance on benchmarks for predicting quantum-related properties of different molecules.
Personal Profile: Shikun Feng is a PhD student in the Department of Computer Science at Tsinghua University, supervised by Professor Yanyan Lan and Professor Weiying Ma. His research focuses on AI4Science, and he has published several papers in related machine learning conferences such as ICML and ICLR. He has also served as a reviewer for KDD and NeurIPS.