Thematic Forums
Forum on Large Language Models to Large Code Models
Hosts: Wanxiang Che
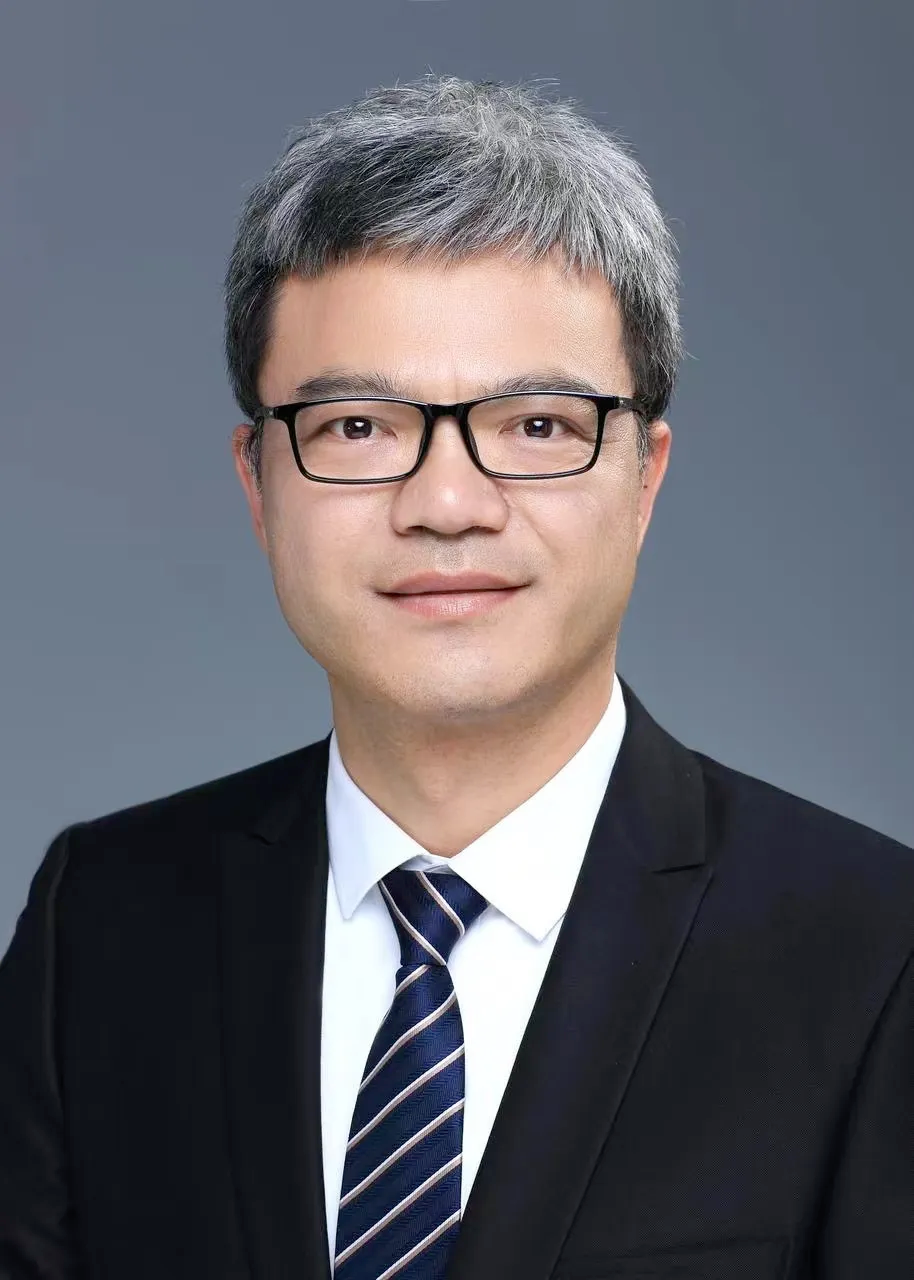
Personal Profile: Che Wanxiang is a Distinguished Professor and Ph.D. Supervisor at the School of Computer Science, Harbin Institute of Technology, and Vice Dean of the Institute of Artificial Intelligence. He is a recipient of the National Youth Talent award, Longjiang Scholar "Youth Scholar," and a visiting scholar at Stanford University. He currently serves as a board member of the Chinese Information Processing Society of China (CIPSC), the Vice Chairman and Secretary-General of the Computational Linguistics Committee, and an Executive Member and Secretary-General of the Asian Association for Computational Linguistics (AACL). He has undertaken several research projects, including key projects of the National Natural Science Foundation of China and the major project of the "New Generation Artificial Intelligence" 2030 initiative. He is the author of the book "Natural Language Processing: Methods Based on Pre-trained Models." He was nominated for the Best Paper Award at AAAI 2013. The language technology platform (LTP) he led in developing has been licensed for paid use by companies such as Baidu, Tencent, and Huawei. In 2016, he won the first prize in the Heilongjiang Provincial Science and Technology Progress Award (ranked 2nd), and in 2020, he received the Heilongjiang Youth Science and Technology Award.
Hosts: Ge Li
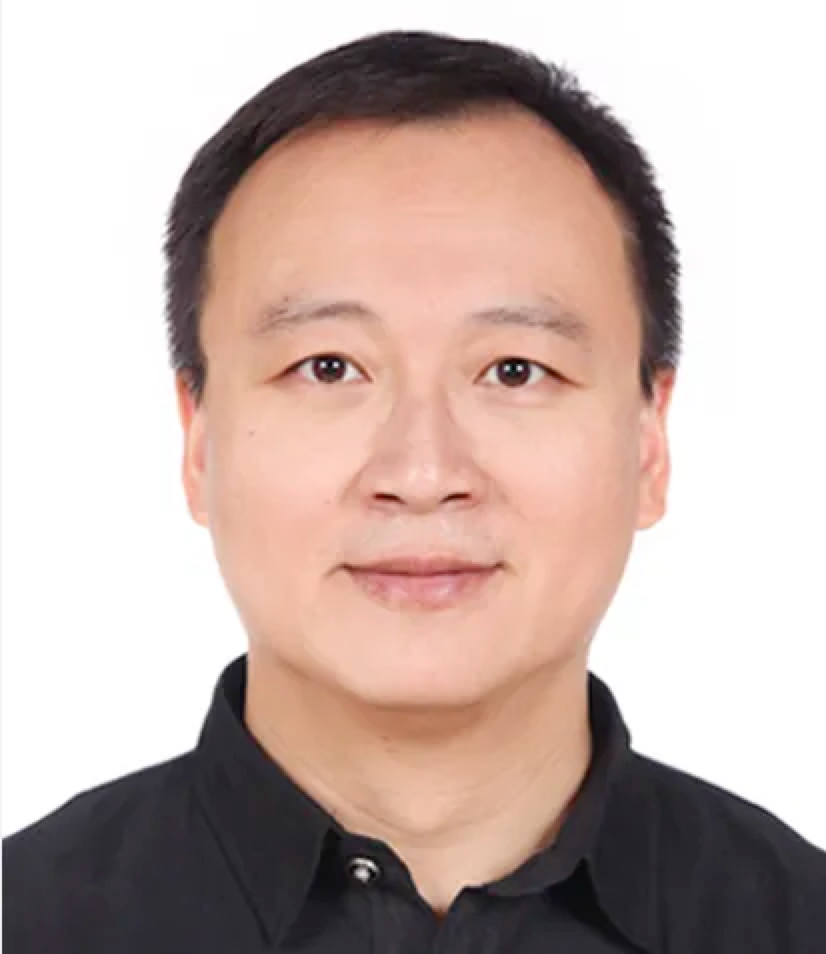
Personal Profile: Ge Li is a Tenured Professor and Ph.D. Supervisor at Peking University, and a National High-Level Talent recipient. He has long focused on research in program understanding, program generation, and deep learning technologies. He is one of the earliest researchers in the world to engage in "program understanding and generation based on deep learning" and has achieved representative results in this field. He has published over 50 related papers in top domestic and international conferences and journals. Many of his papers have been recognized as "groundbreaking work" by international scholars and are widely cited. He has received the ACM Distinguished Paper Award multiple times.
Ge Li has served as co-chair and PC member of program committees for several international conferences in the fields of software and artificial intelligence. He has won the first prize of the Ministry of Education's Science and Technology Progress Award, the first prize of the CCF Science and Technology Invention Award, the second prize of Beijing's Science and Technology Invention Award, and the Zhongchuang Software Talent Award. His teaching courses have been recognized as "National First-Class Offline Courses" and "National First-Class Online Courses" in their first batch of recognitions, and he has won several provincial and ministerial-level teaching awards.
His research and technology transfer achievement, aiXcoder, provides services to major aerospace projects, large enterprises in the finance and IT sectors, and hundreds of thousands of international developers.
Speaker 1: Hui Liu
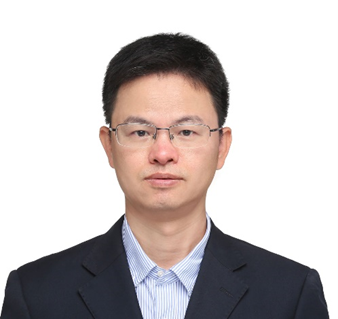
Speaker: Hui Liu
Title: Code Refactoring and Optimization Based on Large Models
Abstract: This talk explores the potential and challenges of large model technology in code optimization, comparing the difficulties and differences between code generation and code optimization based on large models. It analyzes the prospects of large model technology in the field of code optimization. Using software refactoring as an example, it investigates automatic code optimization based on large models, discussing the key technical challenges and potential strategies to address them.
Personal Profile: Hui Liu is a professor at Beijing Institute of Technology and the Secretary-General of the CCF Software Engineering Committee. He has long been engaged in research on software development environments, with over 30 academic papers accepted and published in ICSE, ESEC/FSE, ASE, ISSTA, IEEE TSE, ACM TOSEM, among others. Some of his work has been adopted and integrated into mainstream IDEs like Eclipse. He has received the ESEC/FSE 2023 Distinguished Paper Award, ICSE 2022 Distinguished Paper Award, RE 2021 Best Paper Award, and the IET Premium Award (2016).
Speaker 2: Lin Shi
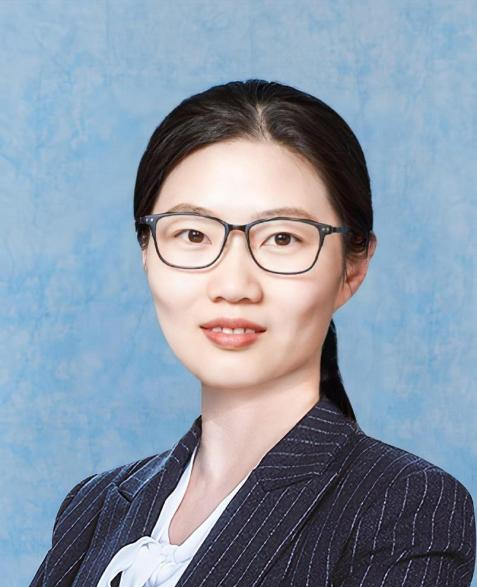
Speaker: Lin Shi
Title: Large Model Code Generation Based on Interactive Requirement Clarification
Abstract: With the significant advancement of AI large models, software development is gradually entering a new era of intelligence. However, it is not easy for developers to write a clear and comprehensive Prompt. Unclear requirement expressions in the Prompt make it difficult for large models to identify the true intentions behind developers, which is one of the major obstacles encountered by large model code generation in practice. This presentation will introduce our latest research in optimizing code generation capabilities, exploring methods based on interactive requirement clarification to help large models better understand user intentions, thereby improving the effectiveness of large model code generation.
Personal Profile: Lin Shi is a professor at Beihang University and a senior member of CCF. His research interests include intelligent software engineering, including intelligent code, intelligent requirements engineering, open source software, trustworthy AI, etc. He has published over 50 papers in high-level international conferences such as IJCAI, ICSE, FSE, ASE in the fields of artificial intelligence and software engineering, and has received three Outstanding Paper Awards: ACM SIGSOFT Distinguished Paper Award (ASE21), two consecutive Outstanding Paper Awards at the International Requirements Engineering Conference (RE21, RE20). He has led and participated in multiple national projects and key cooperation projects with leading enterprises. He also serves as a reviewer for several prestigious international conferences and journals including ICSE, ASE, FSE, and TOSEM.
Speaker 3: Shuai Lu
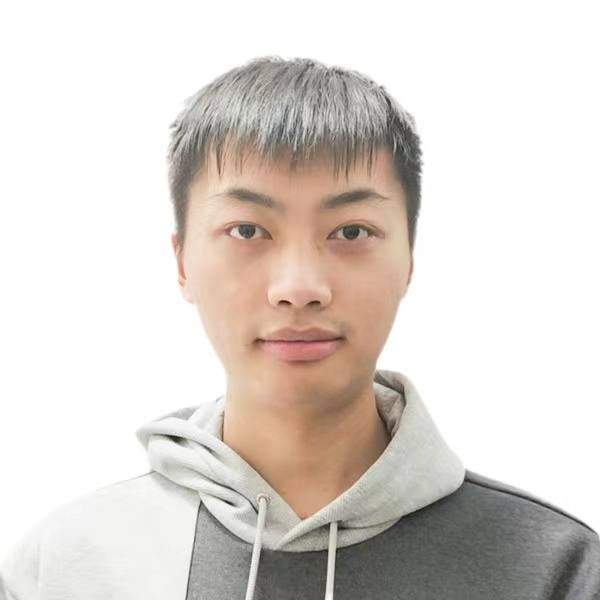
Speaker: Shuai Lu
Title: Trustworthy Code Generation
Abstract: In recent years, large language models have demonstrated remarkable code generation capabilities. However, large models cannot guarantee the accuracy of generated code, especially for complex algorithm implementations or engineering codes, where it is often challenging to generate correct programs in one attempt. To address this issue, the presentation will discuss how to introduce software engineering practices such as program testing or formal verification into the era of large models. Leveraging the powerful generation capabilities of large models, the presentation aims to enhance the credibility of code generation by enabling self-verification within the models. Additionally, it focuses on automating the formal verification process of programs using large models, aiming to verify code reliability from a theoretical proof perspective.
Personal Profile: Shuai Lu is a researcher at Microsoft Research Asia. He graduated from Peking University in 2021, specializing in code intelligence and natural language processing. His research focuses on leveraging deep learning technologies for automating software development to empower programmers. His primary research interests include code autocompletion, code generation, and programming language pretraining models. His research contributions have been published in top AI and software engineering conferences such as NeurIPS, ICLR, ACL, ICSE, FSE, with over three thousand citations on Google Scholar.
Speaker 4: Tao Yu
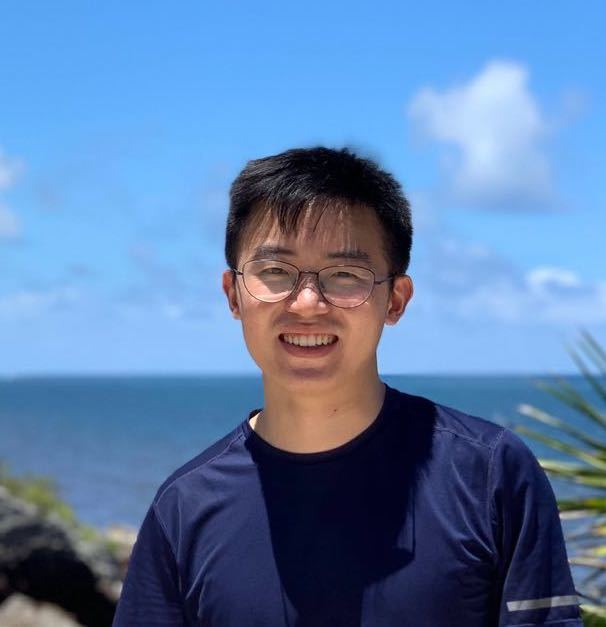
Speaker: Tao Yu
Title: OSWorld: Benchmarking Open-Task Multimodal Agents in Real Computing Environments
Abstract: With the advancements in Visual-Language Models (VLMs), the emergence of autonomous digital agents holds promise to revolutionize human-computer interaction, enhancing accessibility and productivity. These multimodal agents autonomously perform complex reasoning, decision-making, and multi-step action plans across different environments. In this talk, I will primarily introduce OSWorld, a dedicated real computing environment designed to advance the development of agents capable of executing a wide range of digital tasks across various operating systems, interfaces, and applications. I will share insights into cutting-edge VLMs performing open tasks in the OSWorld environment. Additionally, I will discuss some of the latest works in this direction, including fine-tuning retrievers for diverse environment adaptation and enhancing LLM capabilities through tool integration. The presentation will conclude with a discussion on current and future research prospects in this rapidly evolving field.
Personal Profile: Tao Yu is an Assistant Professor of Computer Science at the University of Hong Kong, specializing in natural language processing. He obtained his Ph.D. from Yale University and was a postdoctoral researcher at the University of Washington UWNLP. His research aims to construct language model agents capable of translating language instructions into executable code or actions in real-world environments, including databases, web applications, and the physical world. This forms the core of next-generation natural language interfaces that interact with and learn from the real world through dialogue, facilitating human interaction with data analytics, web applications, and robotic instructions. He has received the Google Research Scholar Award and the Amazon Research Award.
Speaker 5: Qingfu Zhu
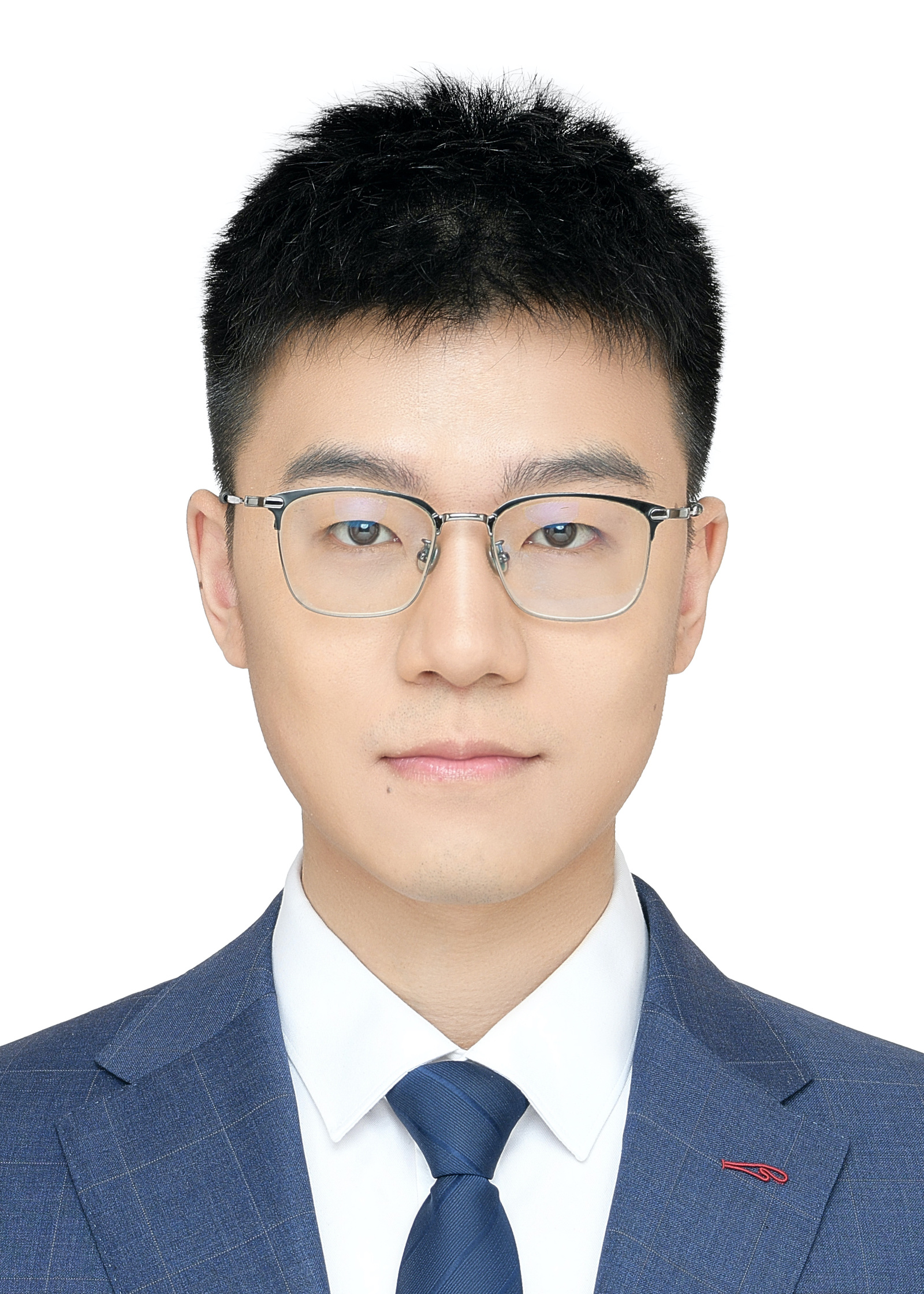
Speaker: Qingfu Zhu
Title: Multilingual Code Models
Abstract: In recent years, the development of code model technology has flourished, leading to the aggregation of more programming language data into large models and thus expanding code generation tasks from single programming languages to multiple ones. Meanwhile, since 95% of the global population speaks non-English native languages, extending code generation tasks to multiple natural languages is equally crucial. This presentation will compare the performance differences of code models among various programming languages and natural languages, introduce methods to enhance performance in low-resource languages, and explore attempts to leverage the multilingual capabilities of code models to improve downstream task performance.
Personal Profile: Qingfu Zhu is an Assistant Professor at Harbin Institute of Technology, with a joint Ph.D. from the University of California, Santa Barbara. His research focuses on natural language processing and code generation. He has published multiple papers in top international conferences in natural language processing, including ACL, AAAI, EMNLP, etc. He has led and participated in several projects funded by the National Natural Science Foundation of China and the "New Generation Artificial Intelligence" Major Program of Science and Technology Innovation 2030.
Speaker 6: Lixing Li
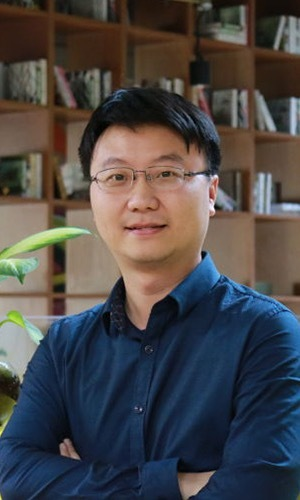
Speaker: Lixing Li
Title: Intelligent Software Development Applications Based on aiXcoder Code Model
Abstract: AI-driven intelligent development based on large models is currently a hot topic and trend in software development technology and tools. There is increasing demand from enterprises for AI-driven software development applications based on code models, yet they face many challenges. The aiXcoder team has been exploring and practicing in this field for over 10 years, pioneering AI-based intelligent development and driving advancements. This presentation will focus on the latest developments of aiXcoder in the field of code models, discussing their explorations and reflections on implementing AI-driven software development technologies and paradigms based on large models.
Personal Profile: Lixing Li is the Chief Operating Officer of aiXcoder, with a Ph.D. in Computer Software and Theory from Peking University/Chinese Academy of Sciences. He has previously served as the algorithm lead of Alibaba Youku Search Team, co-founder and CIO of a medical AI startup, accumulating over 15 years of experience in AI algorithm research and team management. His current responsibilities include leading the research, development, and application deployment of aiXcoder's intelligent software development system.
Forum on Multimodal Large Models
Host: Zhongyu Wei
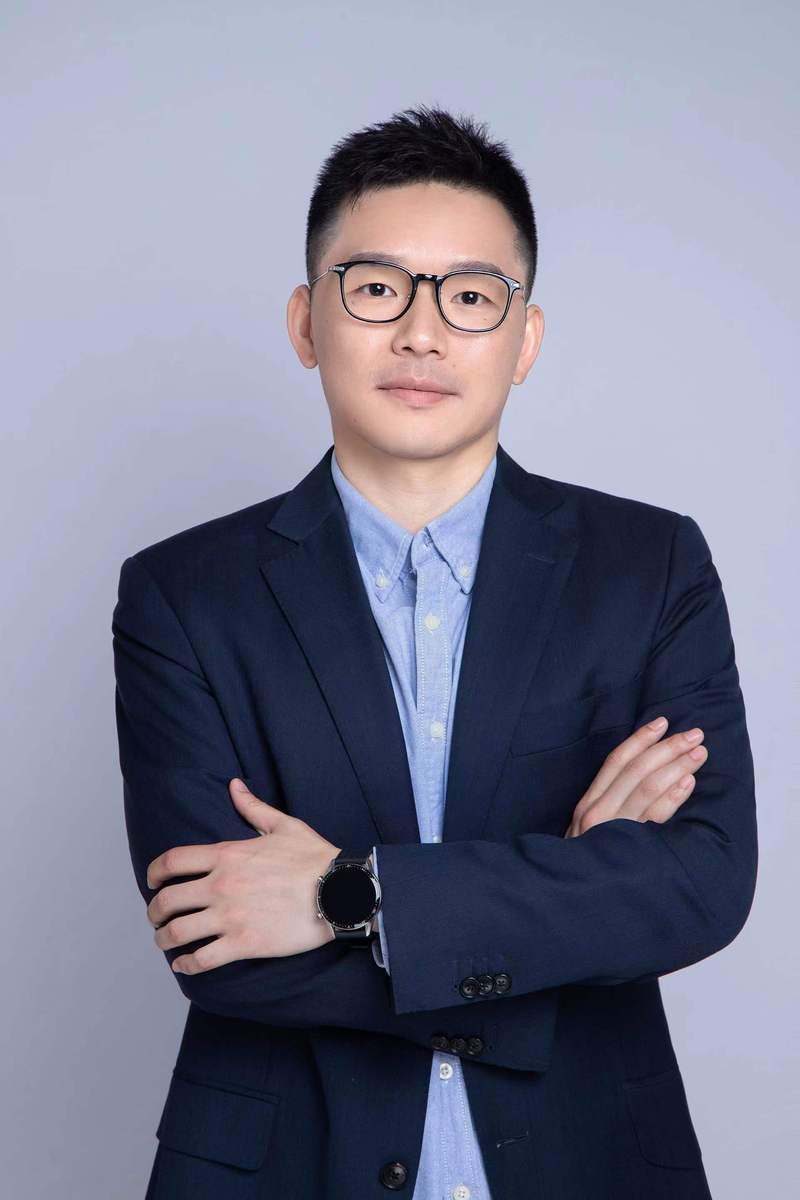
Personal Profile: Zhongyu Wei is an Associate Professor and Ph.D. advisor. He is the head of the Data Intelligence and Social Computing Lab (Fudan DISC) at Fudan University. He obtained his Ph.D. from the Chinese University of Hong Kong and completed his postdoctoral research at the University of Texas at Dallas. He currently serves as the Deputy Secretary-General of the Chinese Information Society's Special Committee on Sentiment Computing, a standing committee member and secretary of the Special Committee on Social Media Processing, and an executive committee member of the Youth Working Committee. He has published over 80 academic papers in international conferences and journals in the fields of natural language processing and artificial intelligence, including CL, ACL, SIGIR, EMNLP, ICML, ICLR, AAAI, and IJCAI. He is a reviewer for several important international conferences and journals and served as the Area Chair for the Multimodal domain at EMNLP 2020 and the Area Chair for Argument Mining at EMNLP 2021. He has received the Shanghai Rising Star Program, Youth Sailing Program, the Chinese Information Society's Emerging Award in Social Media Processing, and the Huawei Technology Outstanding Achievement Award. His main research interests are natural language processing, machine learning, and social media processing, with a focus on multimodal information understanding and generation combining language and vision, argument mining, and interdisciplinary application research.
Host: Benyou Wang
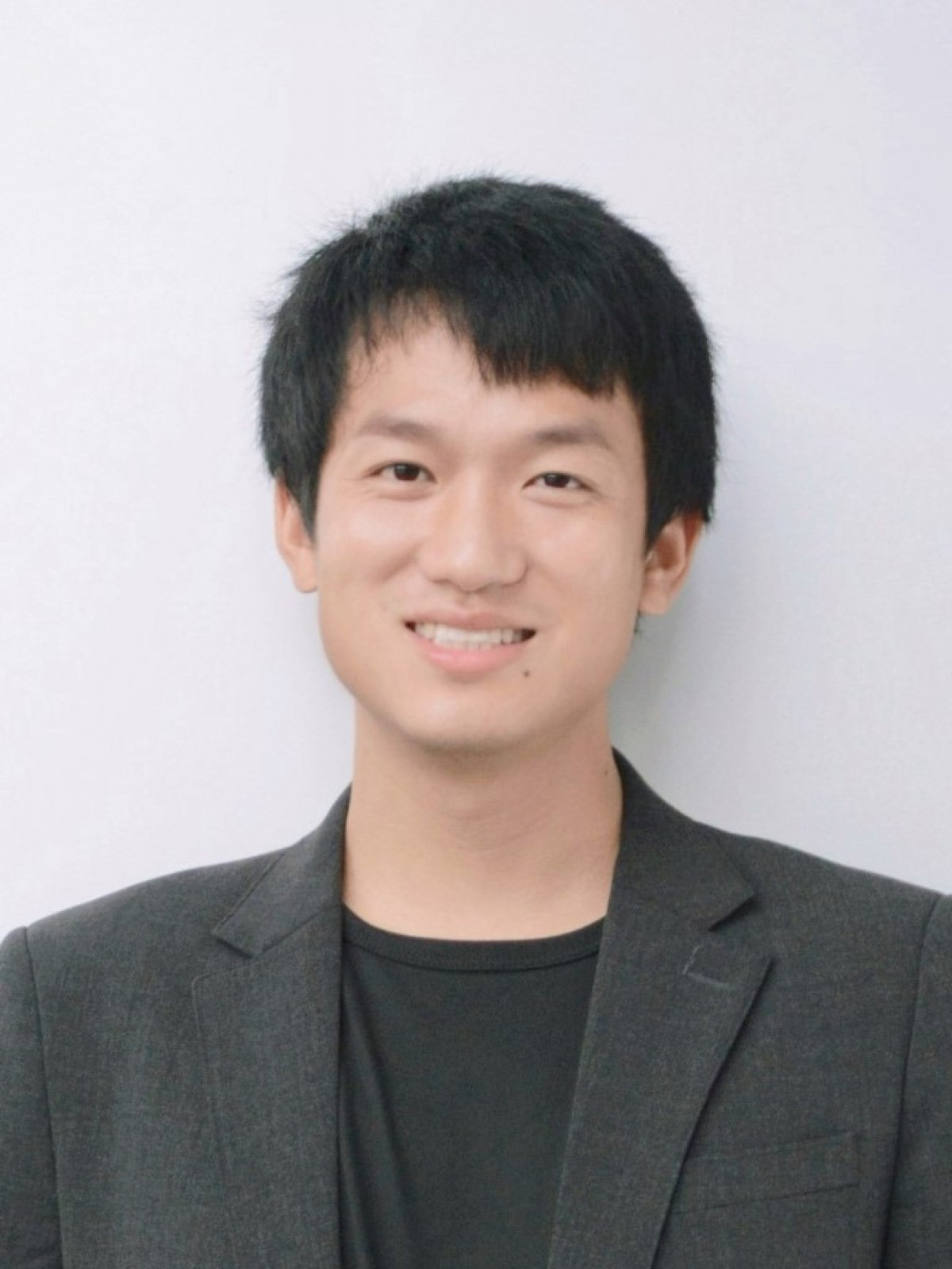
Personal Profile: Benyou Wang is an Assistant Professor at the School of Data Science, The Chinese University of Hong Kong (Shenzhen), and a Research Scientist at the Shenzhen Institute of Big Data. To date, he has received the SIGIR 2017 Best Paper Nomination Award, the NAACL 2019 Best Interpretable NLP Paper, the NLPCC 2022 Best Paper, the Huawei Spark Award, and the Tencent Rhino-Bird Project award. He has also served as the Publicity Chair for NLPCC 2023 and the Website Chair for EMNLP 2023. The large models developed by his research team include HuaTuo GPT for the medical and healthcare vertical and AceGPT, a large language model for Arabic.
Speaker 1: Xinlong Wang
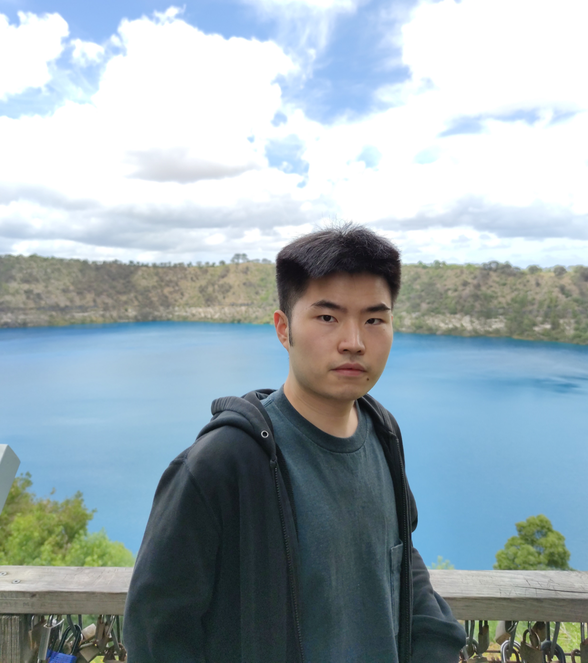
Speaker: Xinlong Wang
Title: Generative Multimodal Models
Abstract: Humans have the ability to easily solve multimodal tasks in context (i.e., with only a few examples or simple instructions), which current multimodal systems struggle to emulate. Large language models have demonstrated powerful language capabilities through generative pretraining, but they still face limitations in handling complex and diverse multimodal tasks. This talk will introduce large-scale generative multimodal models, enabling us to perform multimodal perception and generation tasks with a unified model. It will focus on the latest techniques in multimodal generative pretraining and multimodal context learning, aiming to enhance the model's ability to solve complex perception and generation tasks in multimodal contexts.
Personal Profile: Xinlong Wang is the head of the Vision Model Research Center at Beijing Academy of Artificial Intelligence (BAAI). He received his Bachelor's degree from Tongji University and his Ph.D. from the University of Adelaide, Australia, under the supervision of Professor Chunhua Shen. His research interests include computer vision and foundation models, with recent work covering visual perception (SOLO, SOLOv2), visual representation (DenseCL, EVA), visual context learning (Painter, SegGPT), multimodal representation (EVA-CLIP, Uni3D), and multimodal context learning (Emu, Emu2). He has been awarded the Google PhD Fellowship and recognized as a National High-level Young Talent.
Speaker 2: Ailing Zeng
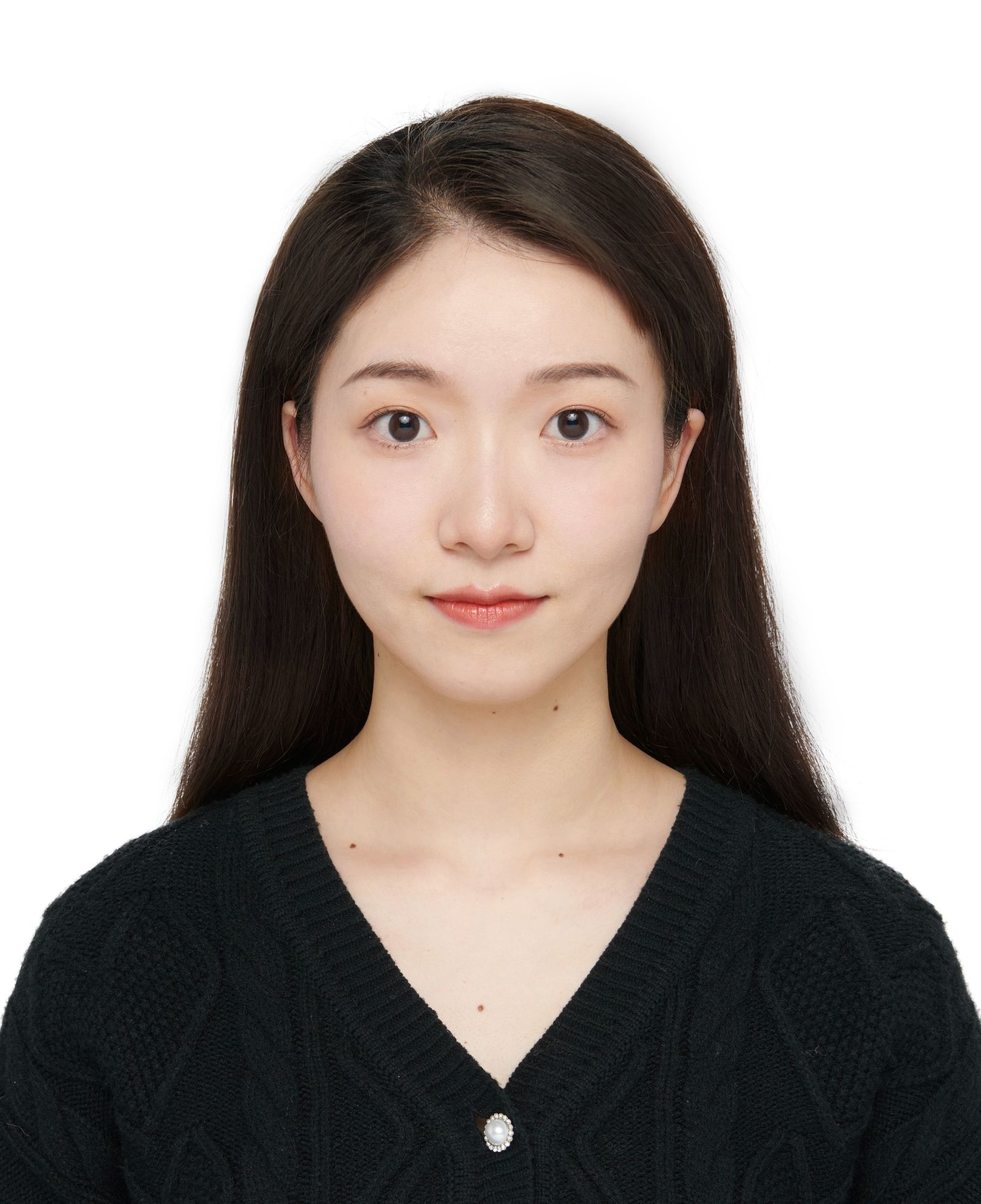
Speaker: Ailing Zeng
Title: Human-Centered Multimodal Perception, Understanding, and Generation
Abstract: Capturing and understanding expressive human actions from arbitrary videos is a fundamental and significant task in computer vision, human-computer interaction, and controllable generation. Unlike high-cost wearable motion capture devices designed for professional users, we have developed a series of markerless motion capture technologies for users of any input image or video, making motion-paired data scalable, low-cost, and diverse. In this talk, I will focus on how to build large-scale human-centered data and benchmarks, including i) automatically annotating multimodal data from internet sources, such as actions, images, videos, text, and audio, ii) understanding human actions from videos using LLM, and iii) controllable 2D to 4D human-centered generation.
Personal Profile: Dr. Ailing Zeng is a Senior Research Scientist at Tencent. Previously, she worked at International Digital Economy Academy (IDEA), leading a team focused on human-centered perception, understanding, and generation. She obtained her Ph.D. from the Chinese University of Hong Kong. Her research aims to build multimodal human-like intelligent agents on scalable big data, particularly large motion models for capturing, understanding, interacting, and generating motions of humans, animals, and the world. She has published over thirty papers at top conferences such as CVPR, ICCV, and NeurIPS, and her first-author paper on long-term time series prediction was among the top three impactful papers at AAAI 2023. Her research outcomes have been transferred to or used in application products, such as DW-Pose in ControlNet ComfyUI for controllable generation and SmoothNet in AnyVision for monitoring areas.
Speaker 3: Bingyi Jing
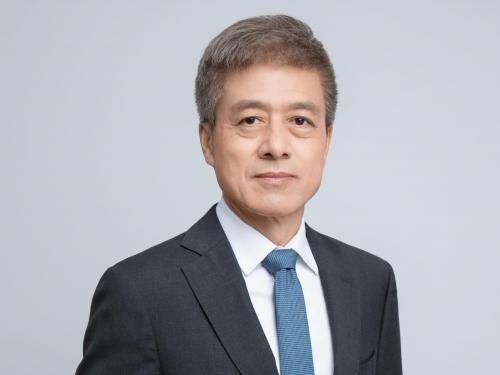
Speaker: Bingyi Jing
Title: How to Achieve Data-Adaptive Selection in Large Model Training?
Abstract: Currently, training large models typically requires massive amounts of internet-scale data. However, the Scaling Law indicates that data quality is crucial for model performance. Therefore, selecting high-quality samples from this massive data becomes a key issue. To address this challenge, we redesigned the data lifecycle in the training process from the ground up. This allows us to introduce different data selection strategies at various stages of training, enabling the model to choose the most suitable data. Additionally, we implemented a learning-based exploration strategy, allowing the model to autonomously select data, further improving training efficiency and model performance. These improvements optimize the data selection process and provide more flexible and intelligent solutions for large model training. This research not only holds theoretical significance but also shows great potential in practical applications, paving the way for future large-scale model training.
Personal Profile: Bingyi Jing is a Chair Professor in the Department of Statistics and Data Science at Southern University of Science and Technology, a National Distinguished Expert, recipient of the Second Prize of the National Natural Science Award, Changjiang Scholar Chair Professor of the Ministry of Education, recipient of the Second Prize of the Higher Education Ministry's Natural Science Award, Fellow of the American Statistical Association (ASA Fellow), Fellow of the Institute of Mathematical Statistics (IMS Fellow), and an Elected Member of the International Statistical Institute (ISI Elected Member). He is the President of the Multivariate Analysis Committee of the Chinese Society of Probability and Statistics and has served as an Associate Editor for seven international academic journals, including Annals of Applied Probability and Journal of Business & Economic Statistics. His research interests include probability and statistics, econometrics, network data, reinforcement learning, and bioinformatics. He has published over 110 papers in top journals and conferences such as Annals of Statistics, Annals of Probability, Journal of American Statistical Association, Journal of Royal Statistical Society Series B, Biometrika, Journal of Econometrics, Journal of Business and Economic Statistics, Bioinformatics, Journal of Machine Learning Research, Science China, and NeurIPS. He has strong collaborations with industry and was awarded the Huawei "Spark Award" in 2023.
Speaker 4: Benyou Wang
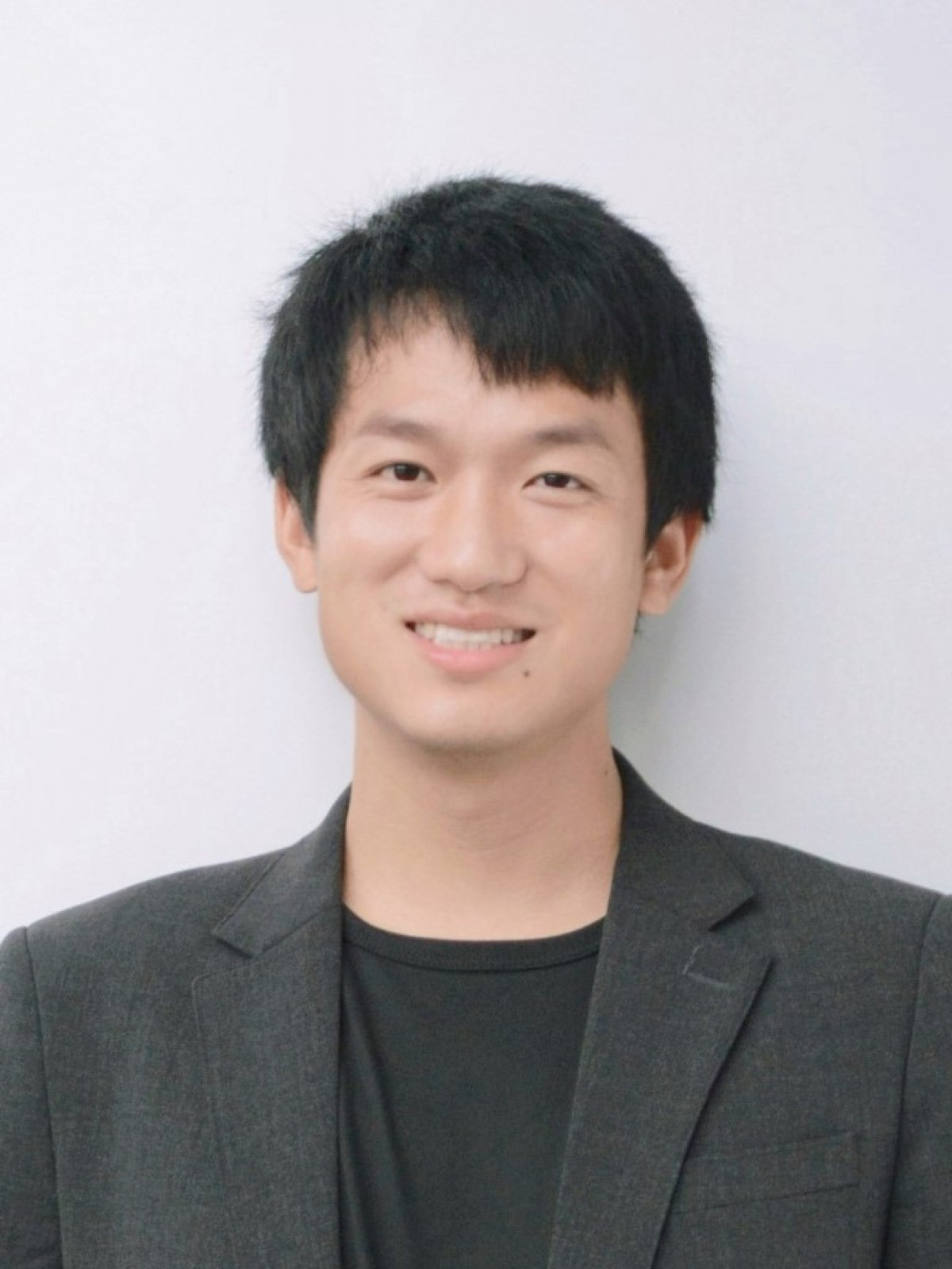
Speaker: Benyou Wang
Title: Multimodal Large Models with Long Contexts
Abstract: The development of multimodal large models heavily relies on data and application scenarios. This talk will first introduce our explorations in data, including the high-quality general multimodal image-text alignment dataset ALLaVA-4V, the supplemental dataset for general long-tail visual knowledge Iceberg-500K, and the medical multimodal knowledge dataset. Furthermore, we will explore multimodal large models with longer contexts and introduce our related benchmark MileBench. Additionally, we will discuss the details of our long-context multimodal large models and their applications in handling high-resolution images and long videos in extended contexts.
Personal Profile: Benyou Wang is an Assistant Professor at the School of Data Science, The Chinese University of Hong Kong (Shenzhen), and a Research Scientist at the Shenzhen Institute of Big Data. To date, he has received the SIGIR 2017 Best Paper Nomination Award, the NAACL 2019 Best Interpretable NLP Paper, the NLPCC 2022 Best Paper, the Huawei Spark Award, and the Tencent Rhino-Bird Project award. He has also served as the Publicity Chair for NLPCC 2023 and the Website Chair for EMNLP 2023. The large models developed by his research team include HuaTuo GPT for the medical and healthcare vertical and AceGPT, a large language model for Arabic.
Forum on Large Model Agents
Host: Chongyang Tao
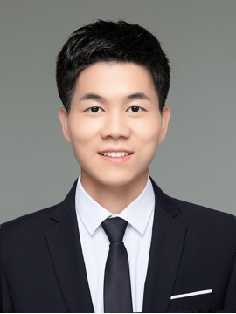
Personal Profile: Chongyang Tao is an Associate Professor at Beihang University. He received his Ph.D. in Science from Peking University in 2020 and later joined Microsoft, where he served as a postdoctoral research scientist and senior research scientist. His research interests include natural language processing and information retrieval, focusing on language models, dialogue systems, efficient knowledge retrieval, among others. He has contributed to the development of Microsoft Xiaobing (Rinna), Bing Chat Assistant, Bing Generation/Search models, and the WizardLM series. He has published over 70 papers in international conferences and journals such as ACL, EMNLP, AAAI, ICLR, SIGIR, and TOIS. He has been awarded the NLPCC Outstanding Paper Award and recognized as an AI 2000 Scholar. He serves as an area chair for conferences like KDD, EMNLP, and CCKS.
Speaker 1: Xu Chen
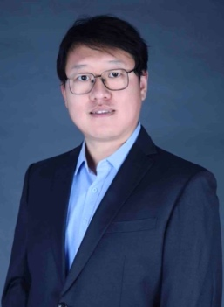
Speaker: Xu Chen
Title: User Behavior Simulation Based on Large Language Model Agents
Abstract: In recent years, Human-centered AI has garnered extensive attention from both academia and industry, with applications such as recommendation systems and social networks greatly facilitating people's lives and productivity. However, a key challenge hindering the development in this field has been acquiring high-quality user behavior data. In this presentation, the speaker will discuss approaches to alleviate this issue from the perspective of LLM-based Agents, and introduce their team's development of RecAgent, an intelligent agent for simulating user behavior based on large language models. This work simulates various behaviors of users in recommendation systems and social networks, where each user acts as an agent capable of engaging in dialogues, posting, searching, self-evolution, etc., within the simulation environment. The speaker will detail the design principles, structural characteristics, usage methods, experimental evaluations of RecAgent, and its potential impacts on the future of Human-centered AI.
Personal Profile: Xu Chen is an Associate Professor at the School of High Ling Artificial Intelligence, Renmin University of China. He received his Ph.D. from Tsinghua University and joined Renmin University of China in 2020. His research focuses on large language models, causal inference, recommendation systems, among others. He has published over 80 papers in top international conferences and journals such as TheWebConf, SIGIR, ICML, NeurIPS, ICLR, AIJ, and KDD, with more than 5800 citations on Google Scholar. He was listed among the top 2% of scientists globally by Stanford University. He co-led the development of the recommendation system toolkit "Bole," co-authored the survey "A Survey on Large Language Model based Autonomous Agents," and constructed the user behavior simulation environment "RecAgent" based on LLM Agents. His research has won the Best Paper Nomination at TheWebConf 2018, the Best Resource Paper Award at CIKM 2022, the Best Paper Nomination at SIGIR-AP 2023, and the Best Paper Award at AIRS 2017. And he has received honors including the CCF Natural Science Second Prize (2nd place), ACM-Beijing Rising Star Award, and CAAI-BDSC Social Computing Young Scholar Rising Star Award. He has led/participated in over ten projects funded by the National Natural Science Foundation of China, the Ministry of Science and Technology, and collaborated with enterprises, with related outcomes implemented in multiple companies. He has been awarded the Huawei "Innovation Pioneer President Award" and the Huawei Excellent School-Enterprise Cooperation Project Award.
Speaker 2: Peng Li
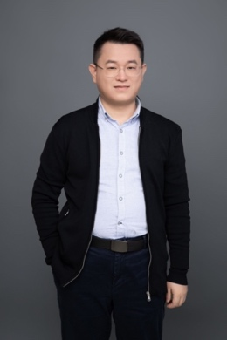
Speaker: Peng Li
Title: Large Model Agents for Open Domain
Abstract: Large models have brought disruptive innovation to the development of artificial intelligence. Effectively utilizing large models to address open-domain issues has become a key topic for the next phase of their development. Recent academic research and industrial practices indicate that intelligent agents based on large models (referred to as large model agents) represent a crucial technological path for extending large models to open domains, with significant research and application prospects. This presentation will share and discuss the main challenges, innovative approaches, and future development directions for large model agents in open domains.
Personal Profile: Peng Li is an Associate Researcher/Associate Professor at the Institute for AI Industry Research, Tsinghua University. His main research interests include natural language processing, pre-trained language models, cross-modal information processing, and large model agents. He has published over 90 papers at major international AI conferences and journals, received the ACL 2023 Outstanding Paper Award, and topped several internationally influential leaderboards, surpassing teams from Google Research, OpenAI, and others. He has led projects such as the key topic under the Science and Technology Innovation 2030 Major Project and the General Program of the National Natural Science Foundation of China. He has served as area chair for important international conferences such as NAACL, COLING, EACL, and AACL. His research outcomes have been applied to high-daily-active-user products from Baidu and Tencent WeChat, achieving significant impact and earning the First Prize of the Qian Weichang Chinese Information Processing Science and Technology Award from the Chinese Information Processing Society of China.
Speaker 3: Xin Gao
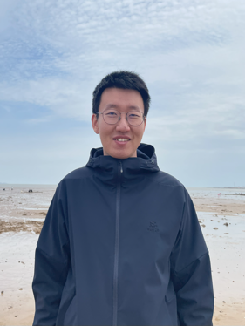
Speaker: Xin Gao
Title: Tool Learning for Large Model-based Agents
Abstract: Research on agents based on large-scale language models is an emerging direction in the field of natural language processing, further advancing the development of general artificial intelligence. This presentation will focus on building the tool invocation capabilities of language model agents, exploring methods for developing foundational tool-use skills in language model agents, and how these can be applied to more downstream tasks.
Personal Profile: Xin Gao is a Distinguished Researcher and Ph.D. Supervisor at the School of Computer Science, University of Electronic Science and Technology of China. His main research areas are pre-trained language models, large model agents, and tool learning. He has published over 40 papers in top international conferences and journals. He currently serves as a member of the Youth Working Committee of the Chinese Information Processing Society of China and a communications member of the Information Retrieval Committee. He is also an area chair and senior program committee member for several top conferences.
Speaker 4: Chen Qian
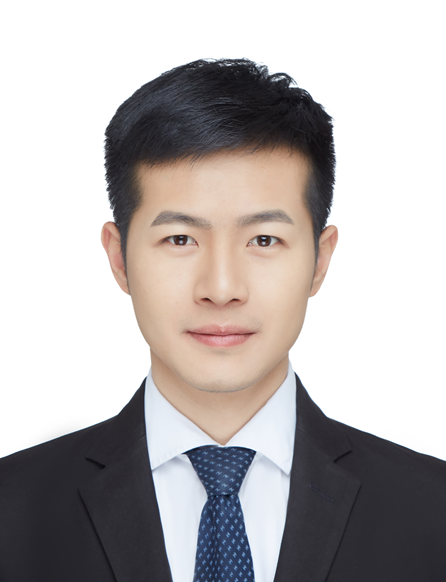
Speaker: Chen Qian
Title: Preliminary Exploration of Scaling Laws for Collaborative Large Model Agents
Abstract: Contemporary large model-driven group collaboration aims to create a virtual team of multiple collaborative agents, autonomously generating complete solutions based on specific task requirements posed by human users through interactive collaboration. This approach achieves efficient and economical reasoning processes, providing new possibilities for automating complex problem-solving. The relevant technology is expected to effectively free humans from traditional labor, realizing the vision of "agents assisting human work." This presentation will cover the key technologies of collaborative multi-agent systems based on large models, including advancements in interaction, collaboration, and evolution, and will preliminarily explore scaling laws for collaboration to guide the construction of efficient multi-agent systems.
Personal Profile: Chen Qian holds a Ph.D. from the School of Software, Tsinghua University, and is currently a postdoctoral researcher at the Tsinghua University Natural Language Processing Lab (THUNLP) and a Shuimu Scholar at Tsinghua University. His main research areas are pre-trained models, autonomous agents, and swarm intelligence. His co-advisors are Professors Maosong Sun and Zhiyuan Liu. He has published several papers as the first author in international academic conferences and journals related to artificial intelligence, information management, and software engineering, such as ACL, SIGIR, ICLR, AAAI, and CIKM. In the field of swarm intelligence, he has led the release of the large language model-driven group collaboration framework ChatDev, the group co-learning paradigm Co-Learning, and the group collaboration network MacNet. He also contributed to the development of the multi-agent platform AgentVerse for task completion and social simulation. ChatDev has gained over 20,000 stars on GitHub, receiving high praise from renowned scholars and enterprises worldwide. Andrew Ng, one of the most authoritative scholars in AI and machine learning, highlighted ChatDev as a representative case in his latest trends and insights on agents published in March 2024.
Speaker 5: Haifeng Zhang
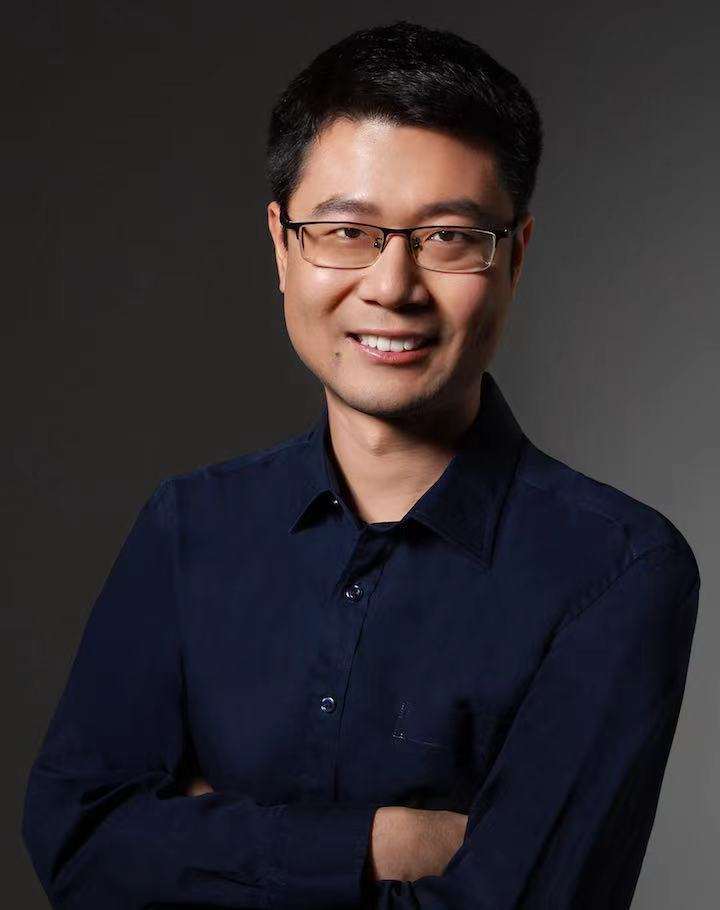
Speaker: Haifeng Zhang
Title: Game Agents Driven by Large Language Models
Abstract: Game agents are a significant thread in the development of artificial intelligence. The advent of large language models provides a new approach to constructing game agents. By using large language models as a foundation and integrating specialized game strategies, it is possible to build game agents with certain general capabilities at a relatively low cost. This presentation will explore the application of this method in various virtual and real-world game scenarios, including StarCraft, soccer games, and socio-economic environments.
Personal Profile: Haifeng Zhang is an Associate Researcher at the Institute of Automation, Chinese Academy of Sciences, and the leader of the Group Decision Intelligence team. He received his bachelor's and doctoral degrees from the Department of Computer Science at Peking University and has conducted postdoctoral research at University College London (UCL). His work focuses on academic research and platform development for multi-agent systems and reinforcement learning. His papers have been published in renowned academic conferences and journals such as ICML, IJCAI, AAAI, AAMAS, and Journal of Software. He leads the development of the "Jidi" intelligent game platform (www.jidiai.cn) at the Institute of Automation, Chinese Academy of Sciences, and undertakes several projects including the National Natural Science Foundation, the Ministry of Science and Technology's "New Generation Artificial Intelligence" major project, and the Chinese Academy of Sciences Pioneer A project. His research has been applied in various fields, including game agents, oil and gas industry chain scheduling, and railway timetable adjustments.
Speaker 6: Ningyu Zhang
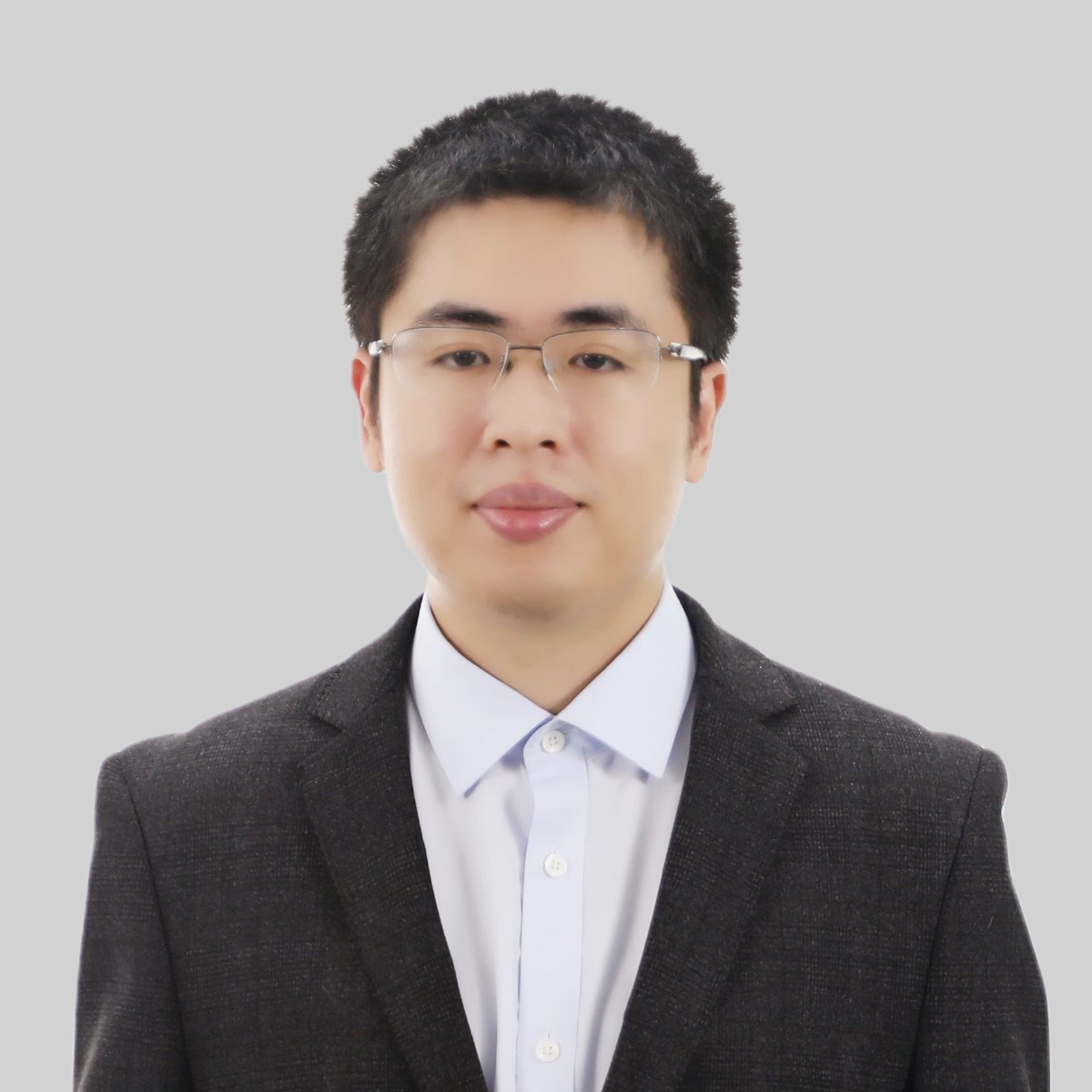
Speaker: Ningyu Zhang
Title: Intelligent Agent Evolution from the Perspective of Knowledge Editing
Abstract: The evolution of large model intelligent agents is a process of enhancing their capabilities through continuous accumulation and optimization of knowledge. In this process, agents improve their knowledge base and decision-making abilities through interaction, learning, and self-improvement. This presentation will explain the process of memory updating and capability evolution in intelligent agents from the perspective of knowledge editing. It will also introduce work related to symbolic and parametric knowledge enhancement for agents. Finally, it will discuss the potential of continuously correcting and expanding the knowledge structure of agents through knowledge editing operations, enabling them to maintain adaptability and flexibility in dynamic environments and better understand complex tasks to improve problem-solving abilities.
Personal Profile: Ningyu Zhang is an Associate Professor at Zhejiang University and a Qi Zhen Excellent Young Scholar at Zhejiang University. He has published numerous papers in high-level international academic journals and conferences, with six papers selected as high-impact papers by Paper Digest and one featured as a Featured Article in a Nature sub-journal. He has led multiple projects funded by the National Natural Science Foundation, the China Computer Federation, and the Chinese Association for Artificial Intelligence. He has received the Second Prize for Scientific and Technological Progress in Zhejiang Province, the IJCKG Best Paper Award/Nomination twice, and the CCKS Best Paper Award once. He has served as Area Chair for ACL and EMNLP, an Action Editor for ARR, and a Senior Program Committee Member for IJCAI. He has led the development of the large language model knowledge editing tool EasyEdit (1.6k).