Multimodal Large Models Forum
Host: Zhongyu Wei
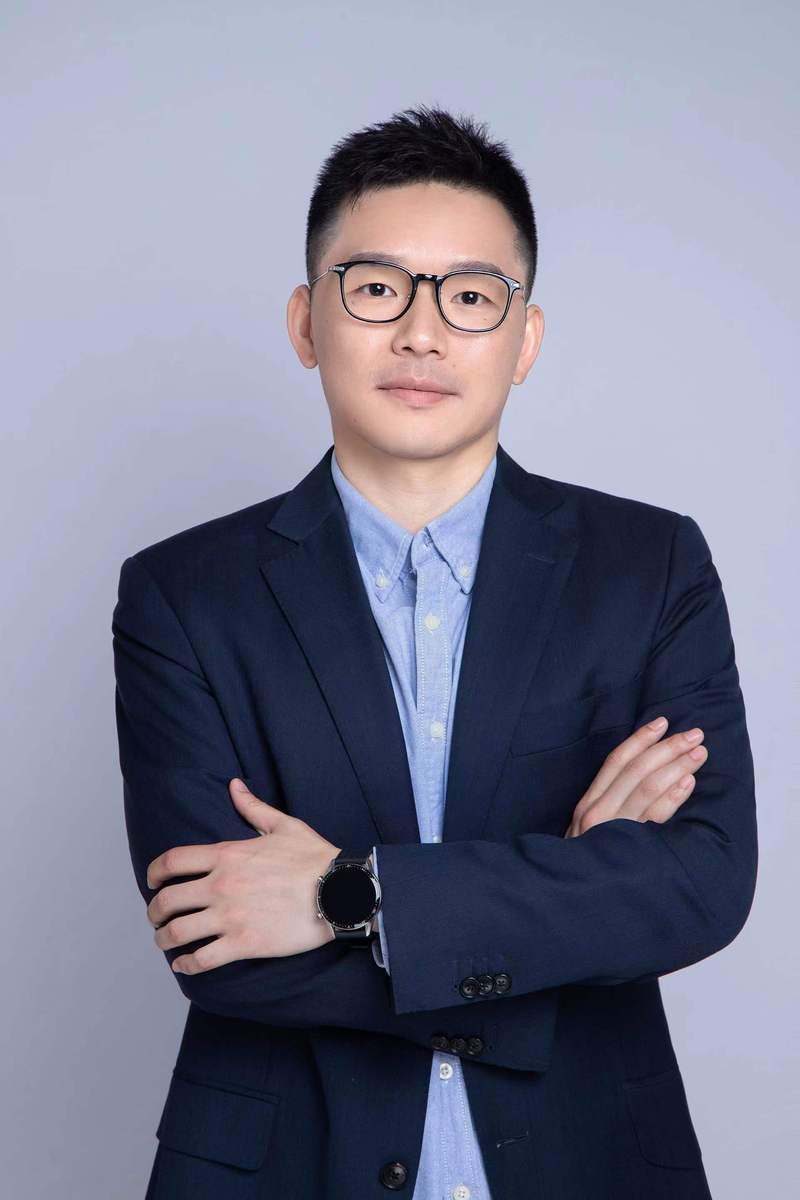
Personal Profile: Zhongyu Wei is an Associate Professor and Ph.D. advisor. He is the head of the Data Intelligence and Social Computing Lab (Fudan DISC) at Fudan University. He obtained his Ph.D. from the Chinese University of Hong Kong and completed his postdoctoral research at the University of Texas at Dallas. He currently serves as the Deputy Secretary-General of the Chinese Information Society's Special Committee on Sentiment Computing, a standing committee member and secretary of the Special Committee on Social Media Processing, and an executive committee member of the Youth Working Committee. He has published over 80 academic papers in international conferences and journals in the fields of natural language processing and artificial intelligence, including CL, ACL, SIGIR, EMNLP, ICML, ICLR, AAAI, and IJCAI. He is a reviewer for several important international conferences and journals and served as the Area Chair for the Multimodal domain at EMNLP 2020 and the Area Chair for Argument Mining at EMNLP 2021. He has received the Shanghai Rising Star Program, Youth Sailing Program, the Chinese Information Society's Emerging Award in Social Media Processing, and the Huawei Technology Outstanding Achievement Award. His main research interests are natural language processing, machine learning, and social media processing, with a focus on multimodal information understanding and generation combining language and vision, argument mining, and interdisciplinary application research.
Host: Benyou Wang
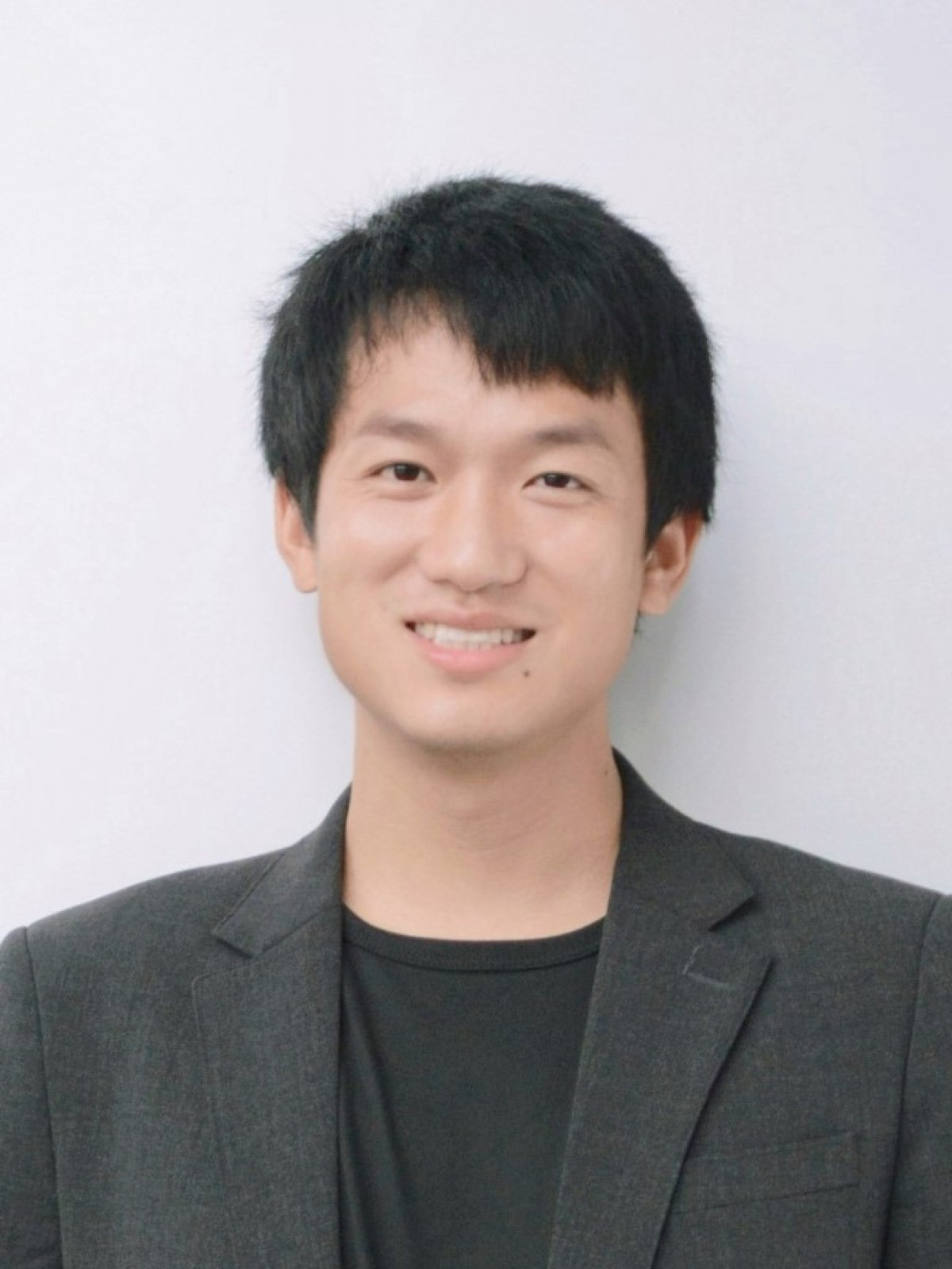
Personal Profile: Benyou Wang is an Assistant Professor at the School of Data Science, The Chinese University of Hong Kong (Shenzhen), and a Research Scientist at the Shenzhen Institute of Big Data. To date, he has received the SIGIR 2017 Best Paper Nomination Award, the NAACL 2019 Best Interpretable NLP Paper, the NLPCC 2022 Best Paper, the Huawei Spark Award, and the Tencent Rhino-Bird Project award. He has also served as the Publicity Chair for NLPCC 2023 and the Website Chair for EMNLP 2023. The large models developed by his research team include HuaTuo GPT for the medical and healthcare vertical and AceGPT, a large language model for Arabic.
Speaker 1: Xinlong Wang
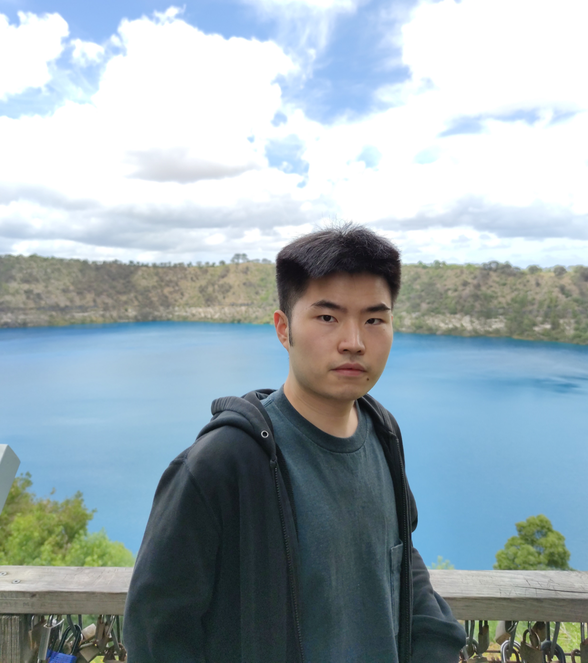
Speaker: Xinlong Wang
Title: Generative Multimodal Models
Abstract: Humans have the ability to easily solve multimodal tasks in context (i.e., with only a few examples or simple instructions), which current multimodal systems struggle to emulate. Large language models have demonstrated powerful language capabilities through generative pretraining, but they still face limitations in handling complex and diverse multimodal tasks. This talk will introduce large-scale generative multimodal models, enabling us to perform multimodal perception and generation tasks with a unified model. It will focus on the latest techniques in multimodal generative pretraining and multimodal context learning, aiming to enhance the model's ability to solve complex perception and generation tasks in multimodal contexts.
Personal Profile: Xinlong Wang is the head of the Vision Model Research Center at Beijing Academy of Artificial Intelligence (BAAI). He received his Bachelor's degree from Tongji University and his Ph.D. from the University of Adelaide, Australia, under the supervision of Professor Chunhua Shen. His research interests include computer vision and foundation models, with recent work covering visual perception (SOLO, SOLOv2), visual representation (DenseCL, EVA), visual context learning (Painter, SegGPT), multimodal representation (EVA-CLIP, Uni3D), and multimodal context learning (Emu, Emu2). He has been awarded the Google PhD Fellowship and recognized as a National High-level Young Talent.
Speaker 2: Ailing Zeng
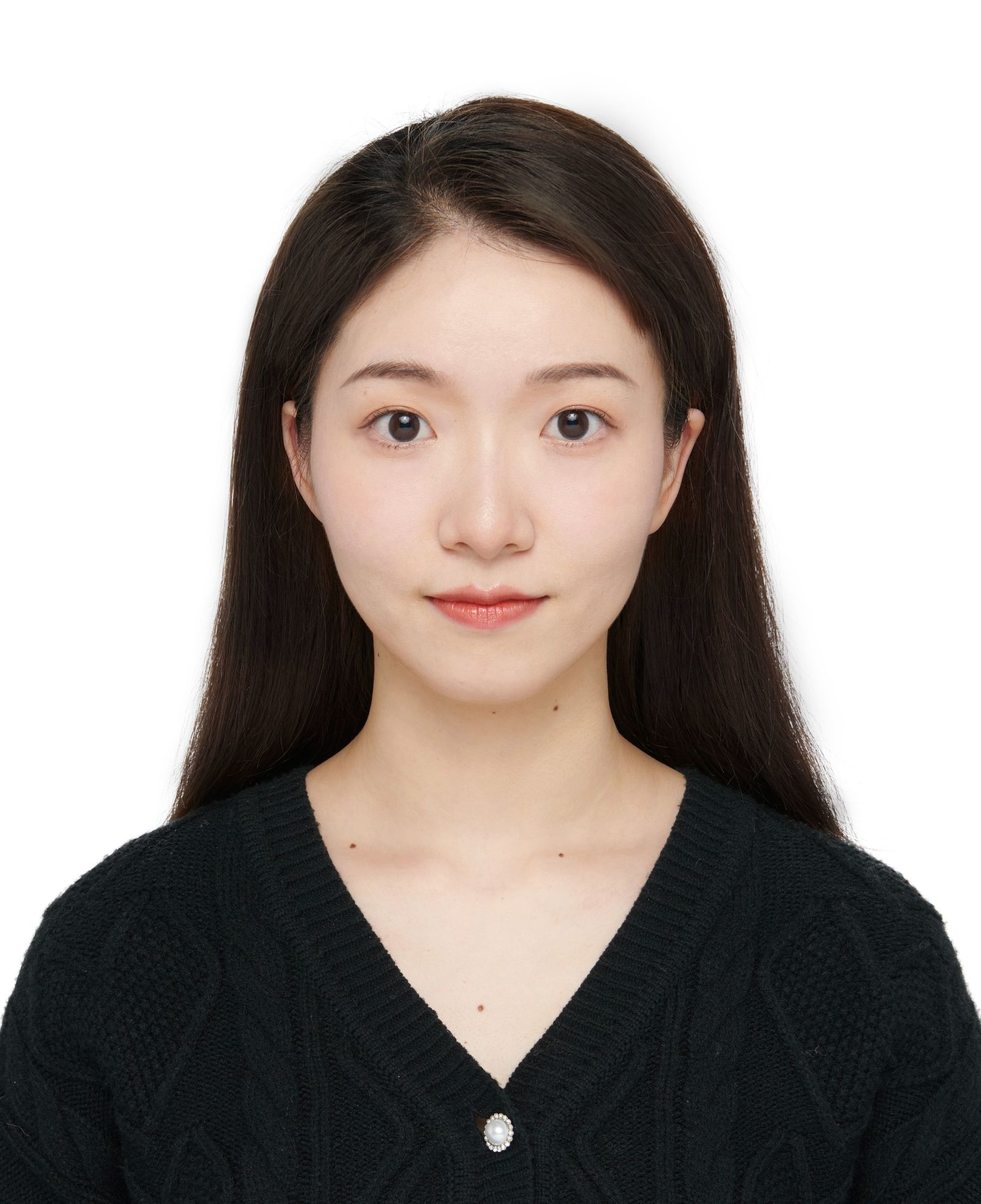
Speaker: Ailing Zeng
Title: Human-Centered Multimodal Perception, Understanding, and Generation
Abstract: Capturing and understanding expressive human actions from arbitrary videos is a fundamental and significant task in computer vision, human-computer interaction, and controllable generation. Unlike high-cost wearable motion capture devices designed for professional users, we have developed a series of markerless motion capture technologies for users of any input image or video, making motion-paired data scalable, low-cost, and diverse. In this talk, I will focus on how to build large-scale human-centered data and benchmarks, including i) automatically annotating multimodal data from internet sources, such as actions, images, videos, text, and audio, ii) understanding human actions from videos using LLM, and iii) controllable 2D to 4D human-centered generation.
Personal Profile: Dr. Ailing Zeng is a Senior Research Scientist at Tencent. Previously, she worked at International Digital Economy Academy (IDEA), leading a team focused on human-centered perception, understanding, and generation. She obtained her Ph.D. from the Chinese University of Hong Kong. Her research aims to build multimodal human-like intelligent agents on scalable big data, particularly large motion models for capturing, understanding, interacting, and generating motions of humans, animals, and the world. She has published over thirty papers at top conferences such as CVPR, ICCV, and NeurIPS, and her first-author paper on long-term time series prediction was among the top three impactful papers at AAAI 2023. Her research outcomes have been transferred to or used in application products, such as DW-Pose in ControlNet ComfyUI for controllable generation and SmoothNet in AnyVision for monitoring areas.
Speaker 3: Bingyi Jing
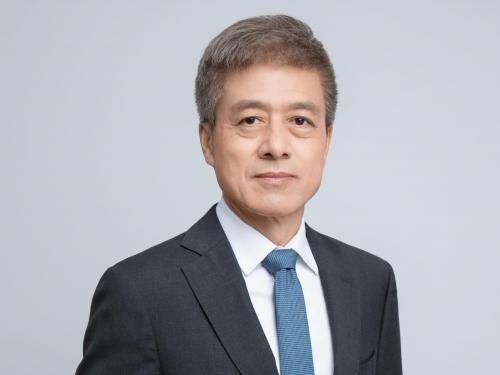
Speaker: Bingyi Jing
Title: How to Achieve Data-Adaptive Selection in Large Model Training?
Abstract: Currently, training large models typically requires massive amounts of internet-scale data. However, the Scaling Law indicates that data quality is crucial for model performance. Therefore, selecting high-quality samples from this massive data becomes a key issue. To address this challenge, we redesigned the data lifecycle in the training process from the ground up. This allows us to introduce different data selection strategies at various stages of training, enabling the model to choose the most suitable data. Additionally, we implemented a learning-based exploration strategy, allowing the model to autonomously select data, further improving training efficiency and model performance. These improvements optimize the data selection process and provide more flexible and intelligent solutions for large model training. This research not only holds theoretical significance but also shows great potential in practical applications, paving the way for future large-scale model training.
Personal Profile: Bingyi Jing is a Chair Professor in the Department of Statistics and Data Science at Southern University of Science and Technology, a National Distinguished Expert, recipient of the Second Prize of the National Natural Science Award, Changjiang Scholar Chair Professor of the Ministry of Education, recipient of the Second Prize of the Higher Education Ministry's Natural Science Award, Fellow of the American Statistical Association (ASA Fellow), Fellow of the Institute of Mathematical Statistics (IMS Fellow), and an Elected Member of the International Statistical Institute (ISI Elected Member). He is the President of the Multivariate Analysis Committee of the Chinese Society of Probability and Statistics and has served as an Associate Editor for seven international academic journals, including Annals of Applied Probability and Journal of Business & Economic Statistics. His research interests include probability and statistics, econometrics, network data, reinforcement learning, and bioinformatics. He has published over 110 papers in top journals and conferences such as Annals of Statistics, Annals of Probability, Journal of American Statistical Association, Journal of Royal Statistical Society Series B, Biometrika, Journal of Econometrics, Journal of Business and Economic Statistics, Bioinformatics, Journal of Machine Learning Research, Science China, and NeurIPS. He has strong collaborations with industry and was awarded the Huawei "Spark Award" in 2023.
Speaker 4: Benyou Wang
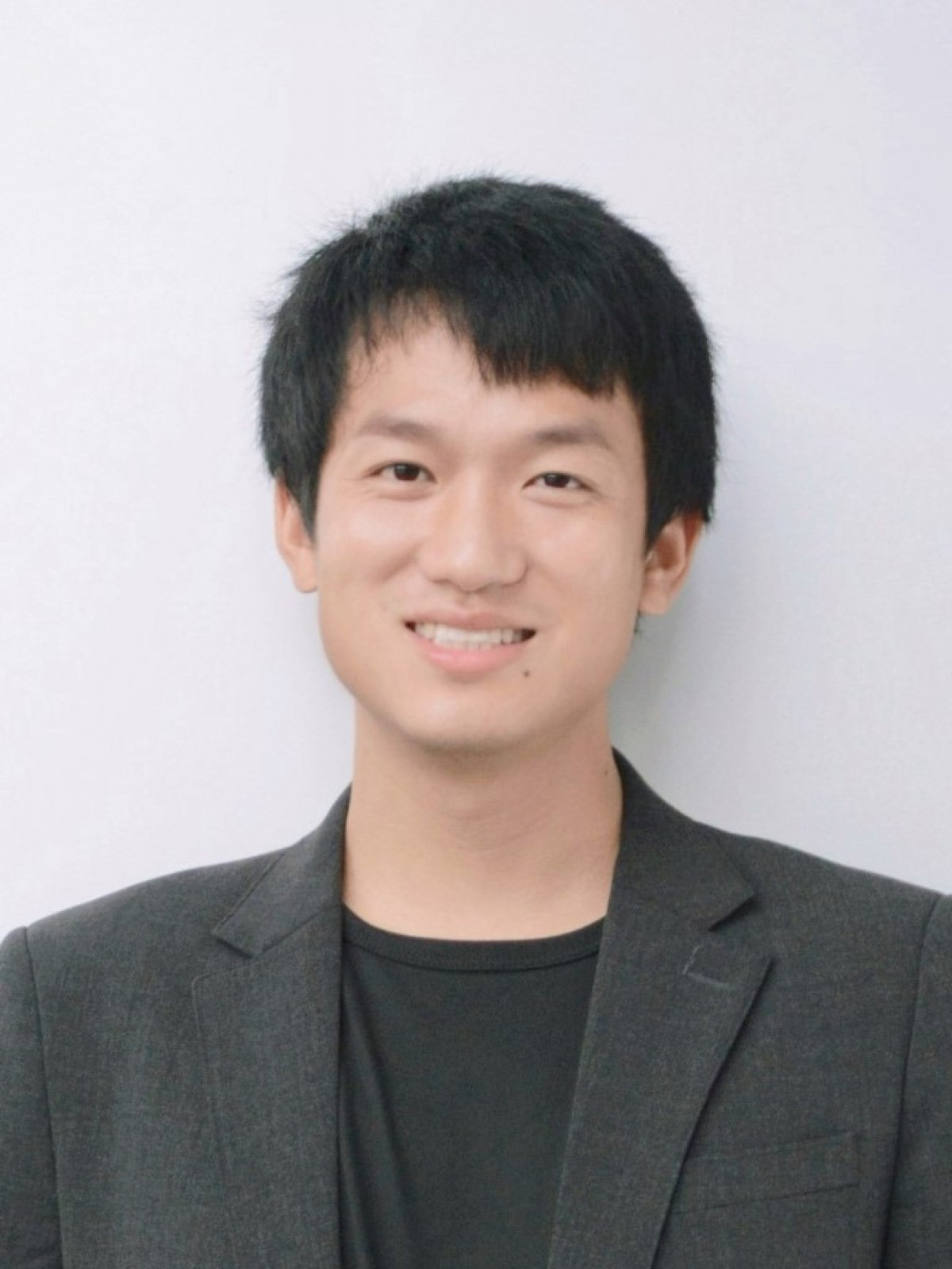
Speaker: Benyou Wang
Title: Multimodal Large Models with Long Contexts
Abstract: The development of multimodal large models heavily relies on data and application scenarios. This talk will first introduce our explorations in data, including the high-quality general multimodal image-text alignment dataset ALLaVA-4V, the supplemental dataset for general long-tail visual knowledge Iceberg-500K, and the medical multimodal knowledge dataset. Furthermore, we will explore multimodal large models with longer contexts and introduce our related benchmark MileBench. Additionally, we will discuss the details of our long-context multimodal large models and their applications in handling high-resolution images and long videos in extended contexts.
Personal Profile: Benyou Wang is an Assistant Professor at the School of Data Science, The Chinese University of Hong Kong (Shenzhen), and a Research Scientist at the Shenzhen Institute of Big Data. To date, he has received the SIGIR 2017 Best Paper Nomination Award, the NAACL 2019 Best Interpretable NLP Paper, the NLPCC 2022 Best Paper, the Huawei Spark Award, and the Tencent Rhino-Bird Project award. He has also served as the Publicity Chair for NLPCC 2023 and the Website Chair for EMNLP 2023. The large models developed by his research team include HuaTuo GPT for the medical and healthcare vertical and AceGPT, a large language model for Arabic.